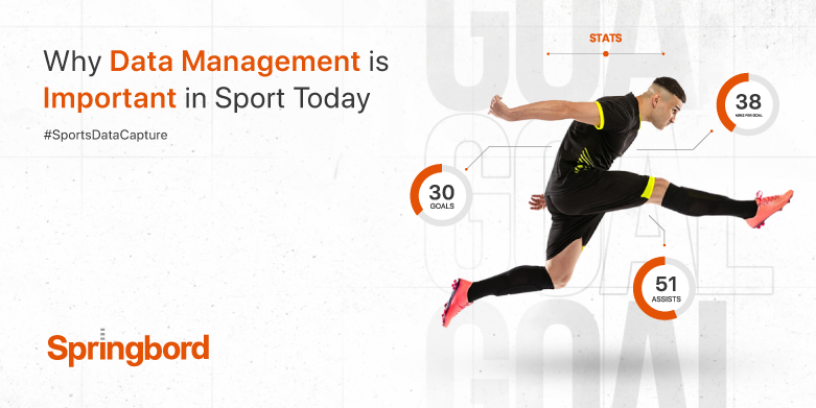
Thanks to technological developments, we now have access to unprecedented data. There is a lot of vital information available for sports teams’ benefit. Many people think of the film “Moneyball” when they consider sports analysis. However, the film provides a superficial look at the field. Sports analysis is used for a wide variety of purposes in the sports industry, including but not limited to increasing profits, boosting individual and team performance, reducing the risk of injury, and more.
This information is precious, but it is useless until it is interpreted and analyzed by human beings. There is a growing need for skilled sports analysts as clubs create departments dedicated only to analyzing data to improve their standing in the league. In other words, teams are employing analytics to get an edge in games. This year should see several noteworthy innovations in the field of technology, thanks to the steady march of progress. Specifically, there have been three developments: integrating data sources to boost competition, disseminating information about why the data is helpful, and developing a new fan experience. Coaches, general managers, agents, scouts, marketers, doctors, and analytics experts are just some of the people who can use this information to do their jobs better. Below are reasons why sports data management is essential.
Injury Predictions
Wearable technology is becoming highly popular in the sporting world, which bodes well for the future of Sports Data Analytics. To better understand how to forecast and prevent injuries, researchers evaluated the effects of the Zephyr BioHarness Wearable Technology on the wearer’s body mass index and total mechanical load, as seen in the graph.
Injured players can be monitored so the training staff can adjust their workloads and get them into conditioning programs if necessary. Logarithmic Regression models based on the Binomial Distribution are frequently employed to estimate the likelihood of player injuries.
Analysts can collect and store Neuromuscular data in a Data Warehouse using various platforms and sophisticated analysis applications. Changes in this data can be monitored throughout the season. Convolutional neural networks can be used to detect anomalies (CNN).
Ratings of Individual Players
The market worth of a player depends on a wide range of factors. The overall buzz surrounding them, the quality of their work, and their reliability are all crucial factors. Whenever a team spends a significant amount of money on a player, they need evidence to back up their decision. When using a data-driven strategy, even the smallest teams can afford to compete at the major league level by acquiring the appropriate players. Scouts need to collect play-by-play data and run it via Machine Learning algorithms to create predictions.
Scouts can learn more about a player’s potential in their current system by using Text-based Sports Data Analytics and Predictive Modeling. For instance, a smaller team may not be able to afford an injury-prone superstar player if they need a leader who will be present for the entire season. It’s crucial to keep in mind that a league’s prominence and status determine the technology level it uses. The National Basketball Association (NBA) uses cameras to capture every second of every game. These cameras record at varying frame rates, each aimed at a unique playing field area.
Machine Learning algorithms can then determine where the ball and players are. However, the National Football League uses chips in the football and the players’ shoulder pads to monitor their movements. Extensive information for forecasting drives, screens, and linemen blocks may be gleaned from comparing the ball’s location and the player’s position. Tracking your jogging pace, resting heart rate, and overall mileage is also possible.
Information like this can assess players’ game performances and track their consistency over the season. This can lead to Predictive Modeling, which is helpful in determining if a player’s success is temporary or indicative of long-term improvement. Likewise, this can aid coaches in deciding which players would thrive under varying circumstances.
Evaluating Ticket Churn
Keeping an existing customer is always preferable compared to the cost of getting a new customer. Logistic Regression techniques are increasingly widely used by sports teams and organizations to analyze Ticket Churn. However, paired T-tests can be used to assess the effect of various marketing initiatives on ticket sales and patron participation.
Teams and clubs can easily estimate the number of season ticket holders who won’t be returning for another year. A team’s success on the field can be used to estimate the return on investment for a club more accurately. No doubt, club supporters will be less likely to attend a game if the team isn’t performing well on the field. We can use predictive data modeling to measure how much of an effect this has.
Conclusion
On the other hand, today’s enterprises typically deal with a massive amount of data constantly changing in format and form. As a result of the increased data volume and Schema differences, businesses will need to invest heavily in developing a data pipeline from scratch for such data. Instead, companies can employ Springbord.
Without manually exporting data or developing the necessary code, Springbord enables you to securely and reliably transfer data from all sources to any destination, including Data Warehouses. You’ll have more time to concentrate on sports data analytics because the data conversion process will be simplified. It is hard to beat as a user-friendly, trustworthy, and secure system.