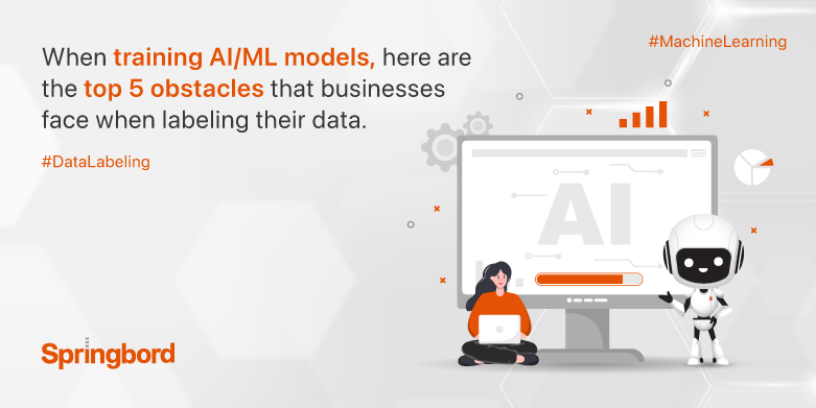
The first step in creating an AI or ML model is the pre-processing phase, also known as data labeling or data annotation. However, Data Annotation can continue after the final AI/ML model has been released, allowing for even greater improvements in accuracy.
Big Data (in the form of pictures, audio, or video files) must be identified by hand and precisely annotated to specify their content during this human-intervened process. After that, the data is fed into AI/ML models so that they can make precise predictions in areas like AI, ML, Computer Vision, and Natural Language Processing.
Machine learning models rely heavily on accurately labeled data. Data efficiency is greatly improved by proper labeling and categorization. Regular scaling up or down of data annotation effort is required due to the ever-shifting demands of machine learning models.
In this article, we will explore 5 obstacles that businesses face when labeling their data.
Five Challenges in Data Labeling
Despite the apparent simplicity of the concept at its core, Data Engineers must address some legitimate concerns.
Five major barriers reduce the efficacy of data labeling, one of which is the poor quality of data that 19% of businesses have reported.
- Insufficient domain knowledge and training data expertise
It takes not only large amounts of big data but also skilled labor, to create and train AI/ML models that produce reliable outcomes. When labeling data, it’s crucial to keep quality and quantity in mind. In the worst case, human error can set off a cascade of other mistakes that will doom the project.
Worryingly, however, domain specialists who are not only data scientists but also have practical knowledge of the technological facets of business applications are sometimes in short supply in many companies.
- Lack of Realistic Objectives, Resources, and Metrics
The cost of implementing AI/ML initiatives has, according to recent Statista research, always been a major worry. The United States is notable because 33% of respondents cited higher costs of data labeling as the primary barrier to integrating AI/ML in business.
Similarly, data annotators can’t work together, the team can’t coordinate their efforts, and nobody will know whether they’ve succeeded unless they have measurable objectives to compare progress against. Not only that, but without Key Performance Indicators, top-level executives would be basing their decisions on misleading data.
- Problems with Employee Management Efficiency
Big data is essential for developing new AI/ML models. The data labeling process still involves a lot of manual labour because it hasn’t benefited from existing AI systems that can at least filter out unstructured data into easily digested bits.
Increasingly large datasets necessitate the employment of large human labour forces to annotate the data for use in artificial intelligence and machine learning systems. This creates yet another difficulty in managing the workforce. Labeling unstructured data to the highest quality is the key to achieving higher accuracy, where organizations are falling short.
- Quality Assurance Procedures That Don’t Work
Both the quality and quantity of big data are beneficial to the fields of Artificial Intelligence and Machine Learning. Increasing the workforce to meet a quantitative goal is simple, but if the quality of the data isn’t up to par, the AI/ML models won’t be trained with the right inputs.
Validating whether data complies with standards is a challenge for data annotation organizations alongside rendering high-quality data. Keep in mind the significance of consistency in making accurate AI/ML model predictions.
- Unable to work along with machines
There is a widespread fear that AI will decimate human jobs. Though this is true to an extent, the outcomes are ultimately attainable through collaborative intelligence, the most prominent example of which is data annotation.
Because of a lack of awareness of the synergistic relationship between humans and AI/ML, businesses aren’t making the most of how human workers can supplement machine output. Machines can improve human leaders by streamlining essential business processes.
Conclusion
Consistent data labeling while ensuring enhanced data security is currently possible, easing concerns about data labeling challenges that can slow down your project.
There has never been a more pressing need for accurate data labeling, and Springbord’s expertise in this area means that they can give you the tools you need to speed up the training of your artificial intelligence and machine learning models without sacrificing accuracy.