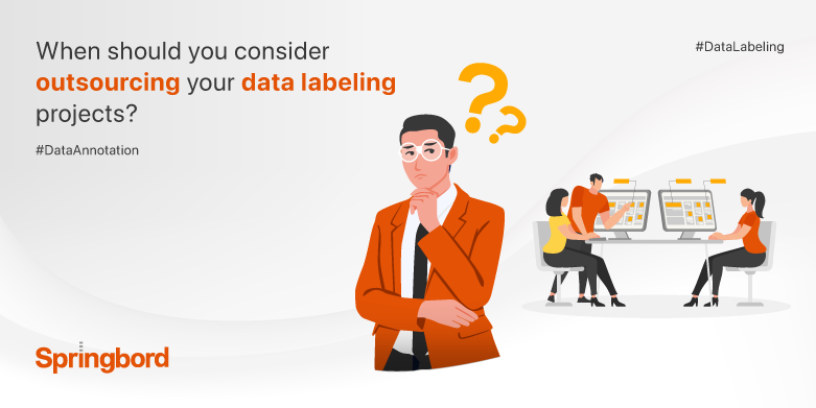
It is common to practise to periodically evaluate the efficacy of current data-labelling practises to ensure they continue to serve the organization.
Cost, time, and a lack of manpower are just some of the difficulties encountered by anyone who has labelled data using in-house teams. some of the warning signs that indicate it might be time to have a professional team handle your data labelling, and explain why doing so will be worth it in the long run.
Categories of Data Labeling
When looking to outsource your data labelling, you have several options to consider.
1. Crowdsourcing
Crowdsourcing, a type of outsourcing in which large numbers of people are tasked with completing small tasks, is rapidly becoming one of the most popular ways to collect data because it is both inexpensive and time-efficient.
Platforms run by a third party typically mediate crowdsourcing projects. In this case, an AI/ML company offers a task on the platform, and then independent employees, and labellers, complete the task.
2. Data Solution Providers
The services they provide, labelling data, are their sole focus. These specialised vendors have extensive experience in data labelling and can meet your needs across the board in your AI/ML project by providing you with high-quality annotations.
These companies may collaborate with your development team to identify best practices and provide quality assurance. They also provide estimates for the amount of data you’ll need and the timeline during which it will be delivered.
3. Business Process Outsourcing (BPO)
Data labelling is an example of a business operation that can be outsourced to a company that is not a specialist in the field. Data entry, customer service, payroll, and data labelling are just some of the many BPO services offered by a wide range of vendors.
These service providers boast an accomplished workforce, which allows them to provide excellent BPS to their clients.
Signs that it’s time to hire data labellers:
1. Cut Expenses
Having a data labelling team in-house requires an investment in resources, including hardware and personnel. Big money will need to be spent on things like staffing, data tagging software, and office space. These costs are reduced when a third-party data labelling provider is used. It will save you the trouble of organising everything and tracking down the right people.
Professionals at data labelling services are well-trained and ready to get to work right away, so they can meet the needs of your dataset promptly. They are probably up-to-date on the most recent developments and resources available in their field. Because of this, they can provide the outcomes you need quickly.
2. Unexpected holdups:
Several factors can reduce an internal team’s performance, including a shift in responsibilities, the need for new skill sets, or a reorganisation of available resources.
Delivery dates can be guaranteed when working with a reliable third-party provider. Thanks to contractual agreements outlining the frequency and quality of data releases.
3. High-Quality Information
An internal team may unwittingly introduce bias that detracts from their work’s precision. The goal of the team classifying the data for AI modelling and machine learning is to give you accurate information.
If you use their annotations and labels, you can rest assured that they are not biased. Thus, the output is of higher quality, which may lead to increased profits for the business.
4. Superior Scalability
Monthly requirements for a dataset are not constant and will change frequently. The in-house team may become overburdened if they also have to handle other responsibilities. In a similar vein, when there are fewer data labelling tasks available, the team is likely to be underworked.
As your company expands, so will your data needs, which can cause inefficiencies that negatively impact your bottom line. By using an outsourcing service, you can save money and time by making better use of your resources.
Conclusion
Today, data labelling services provided by dedicated businesses can make a world of difference when it comes to teaching your algorithms. by cultivating and guiding a multifaceted and dedicated workforce; employing a project group that closely monitors your work and ensures high standards for all annotations.
In addition, your company may be able to make a positive social impact by outsourcing the annotations you need.
An in-house data labelling team could provide more direct oversight, but it would be too much of a hassle to set up and manage. If you’re working on an AI or ML project, Springbord can help you get to the next level by enriching, annotating, and labelling massive amounts of unstructured data. It uses advanced tools, machine learning algorithms, and workflow best practices.