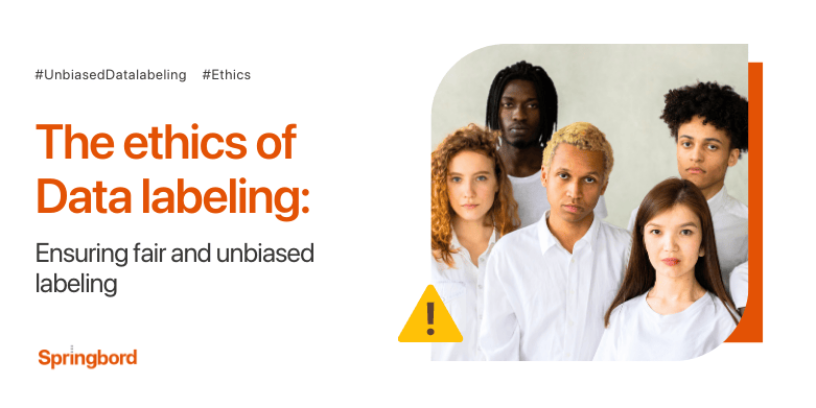
Data labeling, a crucial step in preparing datasets for machine learning models, raises significant ethical concerns. The ethics in data labeling consist of assuring fairness, transparency, and impartiality in the annotation procedure.
The potential biases introduced by labeling, which can lead to discriminatory outcomes and exacerbate existing social inequalities, have ethical implications.
Thus, it is imperative to address the ethics in data labeling to reduce biases, promote impartiality, and encourage the development and deployment of responsible AI systems.
Understanding the Ethical Challenges in Data Labeling
Understanding the ethical challenges in data labeling is essential for ensuring fair and unbiased practices. Critical steps in fostering responsible and ethical data labeling practices include identifying and addressing potential biases, understanding how biassed data affects machine learning algorithms, and investigating ethical concerns, including privacy, consent, and justice.
By prioritizing ethics in data labeling, we can contribute to developing more equitable and trustworthy AI systems.
Potential Biases Introduced Through Labeling:
The integrity and fairness of labeled datasets can be compromised by introducing biases during the data labeling process. Human annotators’ subjective opinions, values, worldviews, and biases can contribute to this issue.
Annotators may be subject to biases based on their preconceived notions about a person’s gender, race, or socioeconomic status, for example. Such prejudices can skew the labeled data, making discrimination and inequality more likely to persist.
Impact of Biased Data on Machine Learning Algorithms:
The performance and outcomes of machine learning algorithms might be negatively impacted by biased data labeled without considering ethical considerations. Algorithms trained using partial data are more likely to reflect and amplify the prejudices and discrimination that exist in society.
This can exacerbate existing inequities by contributing to biased outcomes in healthcare, the criminal justice system, and the hiring process. Biased data can lead to discrimination, inaccurate results, and unjust outcomes for members of underrepresented or marginalized groups.
Ethical Implications: Privacy, Consent, and Fairness:
Data labeling raises important ethical implications beyond the introduction of biases. One such concern is privacy. Privacy is one such issue. When annotating data, it is necessary to handle sensitive information, such as names and addresses, which must be done cautiously.
Individuals must be able to provide informed consent for the labeling and subsequent use of their data; therefore, proper consent processes must be in place.
Labeling data fairly is also an important ethical matter. To be fair, one must act impartially towards all people and all groups. Unfair labeling can be prevented or reduced if many voices are heard, stereotypes are challenged, and new ideas are considered.
Careful consideration must be given to the potential consequences of labeling decisions for various communities, and action must be taken to address any prejudices or unjust treatment that may result.
Ensuring Fairness in Data Labeling
Fairness is a fundamental ethical principle that must be upheld in data labeling. Appropriate data labeling ensures that all individuals and groups included in the labeled datasets are treated fairly.
To achieve fairness, several aspects must be considered, and methods must be implemented to reduce prejudice and increase diversity in representation.
Fairness as a Core Ethical Principle:
One of the most important ethical principles is fairness, which means everyone should be given the same chances and treated equally. In data labeling, fairness is that categorization decisions are not swayed by factors like a subject’s demographics, culture, or socioeconomic status.
To avoid bias and encourage the fair use of labeled data in machine learning applications, fair labeling is essential.
Challenges in Achieving Fairness in Data Labeling:
There are many obstacles to achieving data labeling fairness. Human annotators’ subjective opinions and hidden biases can introduce errors in the labeling process. Furthermore, prejudice and bias in society might play a role in how people are categorized.
One possible cause of skewed results from labeling teams is a need for more diversity of thought. To triumph over these obstacles, we must take decisive action to combat prejudice and advance equality.
Strategies and Techniques for Mitigating Bias and Ensuring Fair Labeling:
- Diverse Labeling Teams: One, more inclusive labeling group: By engaging various perspectives in the labeling process, multi-perspective teams can reduce biases. This variety can help produce more complex and objective categorizations.
- Multiple Perspectives: The influence of personal biases can be mitigated, and fairness can be improved by encouraging collaboration and soliciting feedback from several annotators or experts when labeling confusing or sensitive cases.
Incorporating other viewpoints can achieve a more accurate and fair portrayal of the labeled data.
- Guidelines and Standards: The annotation process can be more reliable by establishing explicit principles and standards for data labeling.
All possible forms of prejudice should be named and dealt with in detail under these regulations. Awareness and sensitivity to issues of fairness can be maintained by ongoing training and instruction on ethical principles.
- Continuous Evaluation and Iterative Improvement: Any biases or unfairness in the labeling process can be identified and corrected through continual review and feedback.
Improving fairness over time is aided by routinely reviewing labeled data, examining any biases, and revising standards and practices based on the findings.
- Auditing and External Oversight: Getting a third party to review your data labeling processes and ensure you follow all the rules is a great way to get fresh eyes on your work.
By analyzing current labeling practices and looking for ways to enhance them, external oversight aids in maintaining accountability, openness, and fairness.
Addressing Bias in Data Labeling
Addressing bias in data labeling is crucial to promoting fairness, accuracy, and ethical integrity in machine learning models. Annotator bias, cultural prejudice, and contextual bias are only a few examples of the potential causes of bias in data labeling.
These prejudices can cause inaccurate portrayals, biased judgments, and the maintenance of existing societal inequalities. Thus, it is crucial to employ tactics and approaches to identify bias in the data labeling process and lessen or eliminate it.
Identification of Common Sources of Bias in Data Labeling:
- Annotator Biases: Human annotators may bring biases into the labeling process, intentionally or unintentionally. Labels applied to data examples may be skewed because of the assigner’s preconceived notions, prejudices, or other biases.
- Cultural Biases: Second, cultural biases might distort how various communities are portrayed in the media because of the weight given to certain factors.
Assumptions, preconceptions, and stereotypes are all forms of cultural bias that influence how people of different genders, races, and socioeconomic backgrounds are classified.
- Contextual Biases: Thirdly, biases might be introduced into data labeling according to the context in which it occurs. The labeling decisions may be biased due to factors such as the labeling instructions, the dataset makeup, or the unique application domain.
Consequences of Biased Labeling on Downstream Applications:
The impact of biased labeling on the performance and fairness of machine learning algorithms and their applications can be significant.
Algorithms taught to make decisions based on skewed data are more likely to make biased judgments and treat people differently. Inaccurate labeling can undermine AI systems’ advantages while reinforcing pre-existing societal biases.
Methods to Detect and Reduce Bias in Data Labeling:
- Clear Guidelines and Instructions: Explicitly addressing potential causes of bias and encouraging fair and unbiased labeling decisions can be aided by providing detailed rules and instructions to annotators. Neutrality, avoiding preconceptions, and treating all people and groups fairly should be emphasized in any guidelines.
- Training and Education: Annotators can improve their ability to recognize and account for their biases through ongoing training and education focused on bias awareness, cultural sensitivity, and ethical considerations.
The effects of bias on data labeling and the implications for subsequent applications should be emphasized in educational settings.
- Diverse and Inclusive Labeling Teams: Third, labeling teams should be diverse and welcoming places where people from all walks of life and experiences can work together to eliminate bias. Labeling decisions that are more fair and balanced can be made by groups that reflect society at large.
- Continuous Evaluation and Feedback: Implementing a system of continuous evaluation and feedback allows for ongoing assessment of labeled data. To correct biases and improve the overall fairness of the labeling process, it is important to regularly evaluate the labeled data, analyze any biases, and provide feedback to annotators.
- External Auditing and Review: Hiring third-party specialists or independent auditors to review the labeling process can help reveal hidden biases and ensure accuracy. Transparency, accountability, and best practices in data labeling are all aided by having an outside party monitor things.
It’s crucial to remember that detecting and eliminating bias can be difficult, as can labeling data with total objectivity. However, data-labeling experts may make substantial strides toward eliminating bias, bolstering justice, and developing more reliable AI systems by adopting the ideas mentioned earlier and methods.
Ensuring Transparency and Accountability in Data Labeling
Transparency and accountability are essential principles in data labeling to promote ethical practices, build trust, and mitigate potential biases or unfair treatment. Labeling decisions, rationale, and any limits or biases in transparent data labeling processes are all made clear.
Mechanisms for accountability ensure that labeling professionals are held to high standards of conduct and that labeling practices can always be evaluated and enhanced.
Importance of Transparency in Data Labelling
Transparency is crucial in establishing trust and credibility in data labeling processes. Organizations and professionals who practice transparency disclose details about their labeling process, including data sources, annotation criteria, and the qualifications of those working on the labeling team.
When made publicly available, end-users, researchers, and regulatory agencies can benefit from assessing labeled datasets’ quality, fairness, and potential biases.
Techniques to Enhance Transparency in Data Labelling:
- Clear Documentation: Data labeling requirements, decision reasons, and potential obstacles should all be documented clearly to ensure openness. Thanks to thorough documentation, stakeholders can learn more about the labeling process, including how conflicts were handled and why certain decisions were made.
- Disclosure of Labelling Methods: Methods of labeling, such as the use of crowdsourcing, professional annotators, or automation, should be disclosed for the sake of openness. It allows stakeholders to evaluate the validity and reliability of the labeled data by learning about the potential biases, limitations, and reliability of each approach.
- Involvement of Stakeholders: Transparency is improved with the participation of all key stakeholders, including the people whose data is being labeled. A more inclusive and accountable labeling strategy includes including these stakeholders in decision-making, soliciting their feedback, and resolving their concerns.
Role of Auditing and Accountability Mechanisms:
- Independent Audits: Data labeling processes can benefit from an external review of their efficacy through independent audits. Ethical standards, possible biases, and recommendations for enhancement are all evaluated during an audit.
Having the labeled datasets independently audited increases trust in their accuracy and reliability.
- Quality Control and Monitoring: Implementing quality control procedures such as routine data quality checks, inter-annotator agreement assessments, and continual monitoring of the labeling process guarantees transparency and responsibility.
Ethical standards can be upheld by monitoring the labeling process and promptly correcting any biases, inaccuracies, or inconsistencies.
- Ethical Guidelines and Standards: Third, moral precepts and regulations: Accountability in data labeling is enhanced by establishing and maintaining clear ethical rules and standards. Anti-discrimination, privacy, consent, and equity are the only topics to be addressed in these rules.
Practitioners’ comprehension and adherence to these rules can be strengthened by consistent training and teaching on ethical considerations in labeling.
To Sum Up
Achieving fair and unbiased labeling in data annotation processes is crucial for developing ethical data practices and unbiased AI systems. The ethical challenges associated with data labeling, such as biases and privacy concerns, underscore the need for transparency, accountability, and equity in these processes.
Springbord Data emerges as a leading provider of high-quality labeling services as organizations and researchers endeavor to overcome these obstacles. With over two decades of experience, Springbord Data offers customized data labeling solutions to satisfy each client’s specific requirements.
They are a trusted partner in promoting ethical data labeling practices due to their expertise in designing custom labels and providing insightful information about customers’ behaviors. Choose Springbord Data for trustworthy and moral data labeling services that contribute to creating impartial AI systems.