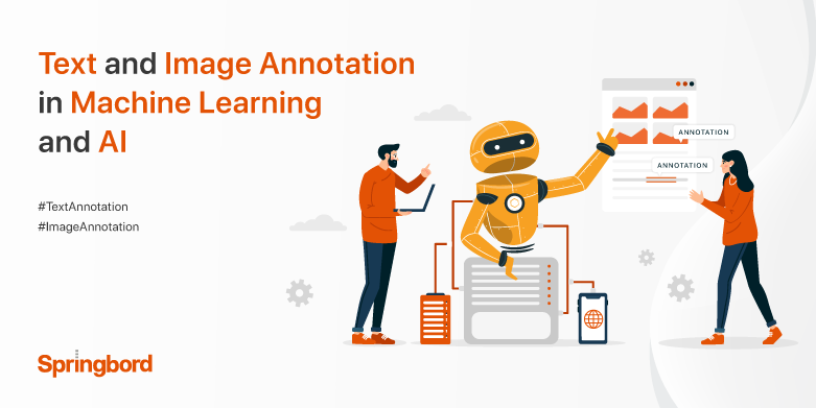
Businesses are embracing Machine Learning (ML) and Artificial Intelligence (AI) for text and image annotation because of the accuracy, speed and comprehensiveness these services provide. In addition, these services eliminate the risks associated with managing data diversity, reducing bias and scaling.
The annotation process begins with marking up a dataset and its characteristics with a metadata tag. For instance, in the case of text annotation involving news reports or journal articles, tags are applied to keywords, phrases, or, in some cases, even personalities. Advanced AI-driven tagging can also be used to identify the context related to a particular piece of content. For instance, it is now possible to tag instances as “positive” or “negative” based on the sentiments expressed. For AI to perform such a feat, it is necessary to ‘educate’ the system to recognize the intent and / or emotion behind the words and the weightage it carries. This is where the algorithms come into play.
AI-driven systems need to be ‘trained’ with algorithms, which require a large number of annotated data to respond based on a model. A majority of today’s digitally savvy companies use chatbots, voice assistants, and even IoT tools to perform transactional communication. These activities, which are often repetitive and mundane, are performed by natural language-based AI systems. This single technology deployment alone would free up abundant resources, which can be gainfully employed in performing core tasks. However, the role of human annotators in designing such AI/ML-driven systems cannot be underplayed. Human annotators provide the necessary feeder data to enable the systems to model their responses based on sentiment, intent, semantics and even relationship.
Similar to tagging in text annotation, AI/ML systems can also tag digital images to describe the visual content. In fact, image annotation has come to play a major role in today’s sporting world, enabling teams and viewers to track the movements of individual players on a ground or sporting arena. This enables coaches and teams to assess, plan and even evaluate their team’s game play. Image annotation includes categorizing and labeling an entire image or the elements in an image. This is done using bounding boxes, which also take into account a wide array of characteristics such as granularity, density and other visual benchmarks. Of course, image annotation tools too require the support of experienced and trained annotators to help ‘model’ the content. Expert annotators play a role in mapping visual features and their semantic meaning to build a model-based learning method for AI tools. On an elementary level, AI tools can also feed on a database of already annotated images to apply the ‘knowledge’ gained. Although these tools ensure faster automation of manual tasks, their advantages are much more than these efficiencies: These tools are a game changer in that they are providing insight and intelligence to the stakeholders to act upon. For instance, intent-driven marketing holds the key for brands to survive. Businesses are capitalizing on AI-driven activities to attract customers with content ‘discoveries’ and ‘experiences’. In fact, smartphone manufacturers are releasing their mobile models with pre-installed AI-driven keypad apps to build their ‘knowledge ecosystem.’
Today, AI/ML-based annotation tools play an important role in leveraging the content ecosystems that businesses have built over the years. At Springbord, our expert teams of annotators have worked with projects of varying complexity across domains. We have access to state-of-the-art toolsets to build an intelligent annotation platform that can withstand the demands of any business requirement for training data models. To know more, get in touch with us for our solutions to your annotation projects.