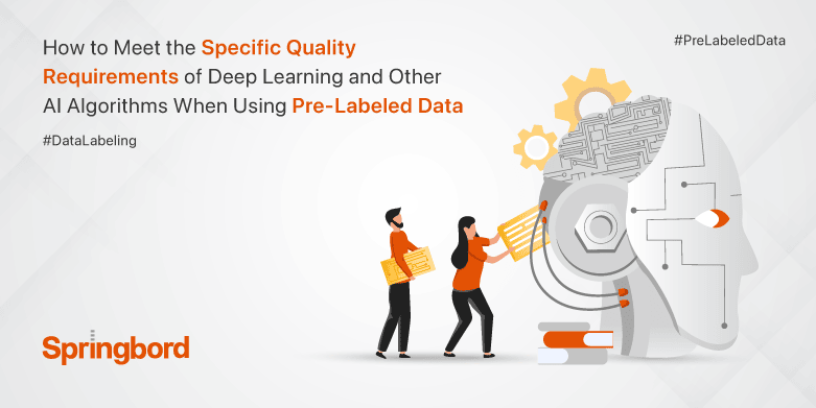
Large amounts of high-quality annotated training data are the foundation upon which successful machine-learning models are constructed. However, gathering this sort of high-quality information can be a time-consuming, tedious, and costly endeavor, which is why some businesses look for ways to automate the data annotation process.
While at first glance automation seems like it would save money, we’ll see that there are potential dangers and hidden costs that could make it more expensive to get to the required annotation quality level and put your project’s timeline at risk.
Pre-labeled Data
Pre-labeled data is the output of an automated object detection and labeling process, during which a specialized AI model creates annotations for the data. Initial steps involve training the model on a subset of ground truth data that has been manually labeled.
When the labeling model has sufficient prior knowledge, it can reliably and automatically assign labels to raw data. Data already labeled may not seem accurate enough for use in a project requiring a high degree of precision. Any endeavour where AI algorithms might affect human well-being, directly or indirectly, falls under this category.
When an organization’s ML model needs to be more well-trained on a specific topic, or when the raw data’s characteristics make it difficult or impossible to automatically detect and label all edge cases, problems can arise with the pre-labeled data. Let’s dive deeper into the challenges businesses might face if they decide to use pre-labeled data.
The price of pre-labeled data may be higher than you expect.
The greater expense of human annotation is a primary motivation for businesses to use pre-labeled data. At first glance, it may appear that automation would result in significant financial savings. It can be expensive to design and fine-tune many artificial intelligence models for pre-labeling purposes to accommodate varied data kinds and scenarios.
As a result, the array of data for which the AI model is developed needs to be sufficiently large for its development to be cost-effective.
Humans are required to annotate certain kinds of data.
There are some types of annotation methods that are hard to replicate using the pre-labeling approach. In general, it is not a good idea to rely solely on auto-labeled data for projects where the model may pose risks to people’s lives, health, or safety. However, automatic annotation tends to produce very low quality when applied to the segmentation of complex objects, especially those with significant boundary inconsistencies.
Furthermore, critical thinking is frequently required while labeling and filing away various items and situations. However, critical thinking will be required to achieve a high-quality level of annotation if the project includes data with a large number of different poses.
Manual annotation is necessary because even the most advanced algorithms of today and the near future cannot think critically.
There Will Be Expenses Connected with Verifying Your Data.
Data pre-annotation algorithms struggle to make sense of projects with numerous moving parts, such as object detection geometry, labeling precision, attribute recognition, and so on. Predictions tend to be of lower quality when the taxonomy and project requirements are more complicated.
From our work with clients, we know that even if an AI/ML team does a great job developing pre-annotation algorithms for cases with inconsistent data and complex guidelines, their results will fall short of the quality level requirement, which is typically at least 95% and can be as high as 99%. The company will have to allocate more resources to manual data validation to ensure a steady stream of high-quality input for the ML system.
Planning the quality validation step and the resources will not only ensure that the project’s quality and deadline are not compromised, but that all necessary information is at hand when it is needed.
There are often concerns and doubts about the pre-labeled data’s accuracy after it has been generated. Inadequately confident results from a labeling model will result in low-quality labels and annotations that cannot be used to effectively train AI/ML systems. Assigning the automatically labeled data to experts so they can verify the annotation quality by hand is a good solution. That’s why the validation stage is crucial: it’s what allows the AI/ML team to rest easy knowing that they’ve reached a high enough quality of data and what gets rid of the delays.
Conclusion
Pre-labeling saves money for businesses, and Springbord knows how important it is to remove any potential for error from high-stakes projects.
We’ve been relieving businesses of the stress that comes with data annotation and quality validation for almost a decade now so that they can focus on creating the most cutting-edge AI solutions.