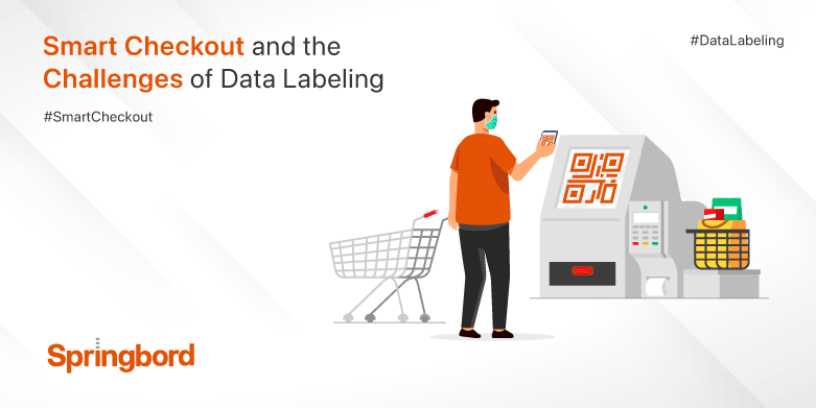
Smart Checkout Annotation is an essential component of modern retail technology. With the increasing demand for automation in the retail industry, the importance of data labeling cannot be overstated.
Data labeling refers to the process of labeling data by assigning relevant labels to images, videos, or text for machine learning purposes. This data is then used to train AI models to automate checkout processes and enhance the customer experience.
In this blog, we will examine the key challenges faced by service providers like Springbord when it comes to data labeling for smart checkout annotation.
Data labeling challenges of cashier-free checkout
Data labeling is a crucial step in the development of AI systems for cashier-free checkout technology. It involves annotating image and video data to train machine learning algorithms to recognize objects in real-world scenarios. The goal of data labeling is to create accurate and diverse training data to enable AI systems to accurately identify objects and make informed decisions. This method, however, is not without its flaws.
One of the biggest challenges of data labeling for cashier-free checkout is the variability of the data. In a real-world setting, objects can appear in different sizes, shapes, colors, and positions, making it difficult for algorithms to recognize them accurately. This variability can lead to incorrect annotations, which can impact the performance of the AI system in real-world scenarios.
Another challenge is the lack of annotated data. Creating annotated data sets can be time-consuming and resource-intensive, and there may not be enough data available to train the algorithms accurately. Additionally, annotators may not have the necessary expertise or experience to label the data correctly, leading to inconsistencies and errors in the data.
Finally, the cost of data labeling is a significant challenge for companies developing cashier-free checkout technology. Outsourcing data labeling to professional annotators can be expensive, and training in-house annotators can be time-consuming and difficult. The high cost of data labeling can make it difficult for small companies and startups to develop AI systems for cashier-free checkout technology, putting them at a disadvantage compared to larger companies with more resources.
Need to lighten your edge caseload?
If you’re facing these challenges in data labeling for cashier-free checkout technology, it’s time to consider partnering with a professional data labeling service provider. At Springbord, we understand the importance of accurate and diverse data labeling for AI systems. Our team of experienced annotators is trained to label data accurately and consistently, and we use state-of-the-art technology to automate the data labeling process, reducing the cost and time required for data labeling.
By partnering with Springbord, you can lighten your edge caseload and focus on developing the best AI systems for cashier-free checkout technology. We’ll take care of the data labeling, so you can focus on the development and testing of your AI systems. With our support, you’ll be able to develop accurate and effective AI systems for cashier-free checkout technology in no time.
Overcome obstacles to smart checkout
Smart checkout systems are becoming increasingly popular as retailers seek to provide a faster, more convenient shopping experience for customers. However, the development and deployment of these systems can be hindered by several data labeling challenges. These challenges include:
1. Unusual or uncommon object shapes:
Some products and objects may have unique shapes or features that are difficult to accurately annotate. This can result in incorrect labels and decreased system performance.
2. Inconsistent lighting conditions:
Lighting conditions can greatly affect the visibility of objects in images, making them difficult to label accurately. This can lead to errors in the data labeling process and negatively impact the system’s performance.
3. A large number of images:
Labeling a large number of images can be a time-consuming and tedious task, especially when done manually. This can result in increased costs and longer project completion times.
At Springbord, we have the expertise and experience to overcome these obstacles and provide our clients with accurate, high-quality data labeling services for their smart checkout systems. Our data annotators have a deep understanding of the challenges involved in smart checkout data labeling and are trained to accurately label even the most complex images.
Conclusion
In conclusion, data labeling is a critical component in the development of smart checkout systems, but it can also be a challenging and time-consuming task.
At Springbord, we have the expertise and experience to overcome the challenges of data labeling and provide our clients with high-quality, accurate results.
With our help, our clients can improve the efficiency and performance of their smart checkout systems, providing a faster, more convenient shopping experience for their customers.