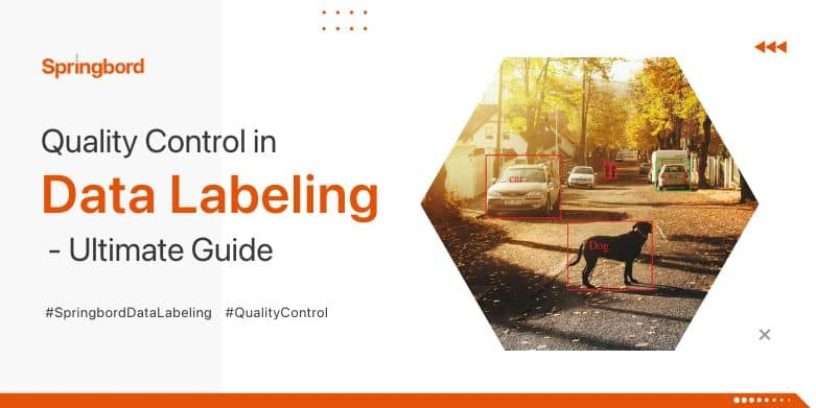
As data becomes more integral to businesses, ensuring the accuracy and reliability of that data becomes paramount. However, data is often not collected in a format that is usable for machine learning algorithms.
This is where data labeling comes in. Data labeling is the process of manually assigning labels to data so that algorithms can learn from it. However, without proper quality control measures in place, data labeling can lead to costly errors and inaccuracies.
At Springbord, we understand the importance of ensuring high-quality labeled data. We provide a data labeling service that helps our clients get the most out of their data. In this ultimate guide, we will discuss the best practices and cutting-edge technologies for quality control in data labeling.
Best Practices for Quality Control in Data Labeling:
- Clear Instructions: The first step in quality control for data labeling is to provide clear instructions. Clear instructions ensure that the data labelers understand what they are supposed to do and how they are supposed to do it. Ambiguous instructions can lead to inconsistent labeling, which can make it difficult for algorithms to learn from the data.
- Training: The second step in quality control for data labeling is to provide training to the data labelers. Training can include the instructions mentioned above, as well as examples of good and bad labeling. This training ensures that the data labelers have a clear understanding of what is expected of them.
- Labeling Consistency: Consistency is critical in data labeling. If multiple labelers are working on the same dataset, they must label the data consistently. Inconsistencies can make it difficult for algorithms to learn from the data and can lead to errors and inaccuracies.
- Quality Assurance: Quality assurance is the process of checking the labeled data for errors and inconsistencies. Manual quality assurance involves reviewing a sample of the labeled data to ensure that it meets the quality standards. Automated quality assurance involves using software tools to check the labeled data for errors and inconsistencies.
- Feedback: Finally, feedback is essential in quality control for data labeling. Feedback helps the data labelers understand where they are making mistakes and how they can improve. Providing feedback also helps to maintain consistency in labeling and ensures that the labeled data is of high quality.
Cutting-Edge Technologies for Quality Control in Data Labeling:
- Active Learning: Active learning is a machine learning technique that can reduce the amount of labeled data needed for a task. Active learning works by selecting the most informative data points for labeling, instead of randomly selecting data points. This can save time and money while improving the accuracy of the labeled data.
- Semi-Supervised Learning: Semi-supervised learning is a machine learning technique that combines labeled and unlabeled data. Semi-supervised learning can be used to reduce the amount of labeled data needed for a task while improving the accuracy of the labeled data.
- Human-in-the-Loop: Human-in-the-loop is a machine learning technique that combines the power of machines with human expertise. Human-in-the-loop can be used to improve the accuracy of labeled data by having humans check and correct the machine-generated labels.
In addition to the best practices and cutting-edge technologies discussed above, there are several other factors to consider when implementing quality control measures for data labeling.
One such factor is the choice of data labelers. It is essential to choose data labelers who are experienced, knowledgeable, and have strong attention to detail. The labelers should also be trained on the specific task they are assigned and have a clear understanding of the labeling guidelines.
Another factor to consider is the type of data being labeled. Some types of data may require more stringent quality control measures than others. For example, medical or financial data may require additional privacy and security measures, as well as specialized labeling guidelines.
Finally, it is important to have a system in place for tracking and managing labeled data. This system should allow for easy tracking of who labeled the data when it was labeled, and any quality control measures that were taken. Having a centralized system for managing labeled data can help ensure that the data is consistent, accurate, and reliable.
Quality control in data labeling is critical for ensuring the accuracy and reliability of labeled data. By following best practices, leveraging cutting-edge technologies, and considering factors like the choice of labelers and the type of data being labeled, businesses can ensure that their labeled data is accurate and reliable. At Springbord, we provide a data labeling service that helps our clients get the most out of their data. Contact us today to learn more about our services and how we can help you with your data labeling needs.
Conclusion:
In conclusion, quality control in data labeling is essential for ensuring the accuracy and reliability of labeled data. At Springbord, we understand the importance of high-quality labeled data and provide a data labeling service that helps our clients get the most out of their data.
We have discussed the best practices and cutting-edge technologies for quality control in data labeling. By following these best practices and leveraging cutting-edge technologies, businesses can ensure that their labeled data is accurate and reliable.