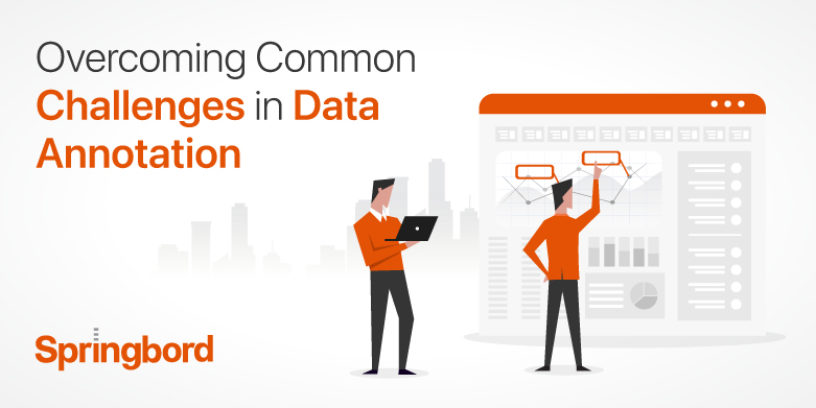
Introduction
In the rapidly advancing field of artificial intelligence and machine learning, the role of data annotation is pivotal. By labeling or tagging data such as images, text, and videos, businesses can train algorithms to identify patterns and make predictive analyses, turning raw data into strategic assets.
As businesses increasingly rely on AI to enhance operational efficiency and customer engagement, the quality of data annotation directly influences the effectiveness of AI applications. At Springbord, we recognize the transformative power of expertly managed data services.
This blog will delve into the common challenges of data annotation and explore advanced strategies and solutions that businesses can adopt to enhance the accuracy and efficiency of their AI models, thereby securing a competitive edge in the digital era.
Overview of Data Annotation
Data annotation is the backbone of machine learning and artificial intelligence (AI), crucial for the development and refinement of AI models.
This process is fundamental for various applications, from autonomous vehicles to personalized shopping experiences, as it helps AI understand and interact with the world around it.
Relevance to Business Owners
For business owners, high-quality data annotation is not just a technical necessity; it’s a strategic asset. It directly influences the accuracy, efficiency, and capability of AI systems, impacting everything from operational efficiency to customer engagement. Businesses that invest in robust data annotation processes can expect to see substantial returns in the form of improved AI performance and enhanced competitive edge.
Whether it’s enhancing customer experience or refining predictive models, Springbord’s expertise in managing and annotating diverse datasets ensures that businesses not only meet but exceed their technological and operational goals.
Challenge: Scalability and Management of Large Data Sets
Scaling data annotation to manage large datasets effectively presents significant challenges, especially as businesses increasingly depend on artificial intelligence for critical insights and operations.
Problem Outline
As businesses increasingly leverage AI to drive innovation and efficiency, the need for data annotation scales accordingly. However, scaling data annotation efforts to keep pace with growing data volumes presents significant challenges. High-quality data annotation becomes more complex and resource-intensive as the amount and variety of data increase. This can lead to increased costs, longer project timelines, and potential compromises in data quality.
Solutions
- Automation Tools:
To tackle scalability in data annotation, integrating semi-supervised learning algorithms can greatly reduce manual efforts by using both labeled and unlabeled data, thus boosting annotator productivity by allowing them to focus more on refining pre-labeled data.
- Workforce Management:
Efficient management of a scalable annotation team is crucial for handling large data volumes. Employing a hybrid model that combines in-house expertise with crowd-sourced efforts provides necessary flexibility, while rigorous training and continuous performance assessments ensure high-quality annotations across projects.
At Springbord, our expertise in handling large datasets ensures that we not only manage the volume but also maintain the integrity and quality of the data throughout the annotation process.
Challenge: Ensuring Data Quality and Accuracy
Ensuring data quality and accuracy in annotation is crucial for the reliability of machine learning models, necessitating comprehensive quality control measures and consistent training for annotators.
Problem Outline
Maintaining high-quality and accurate data annotations is crucial for the reliability of machine learning models, requiring rigorous quality control measures and continuous annotator training to manage data consistency and reduce errors.
Solutions
- Rigorous Quality Control
Implementing strong quality control systems ensures data annotation accuracy. This involves:
- Double Annotation and Adjudication Processes: Multiple annotators assess the same data points, with adjudication to resolve discrepancies. This method reduces biases and increases data reliability.
- Regular Quality Audits: Frequent reviews and audits detect and correct errors early, preventing data quality drifts and inconsistencies.
Advanced Training
Continual training and feedback for annotators are crucial to enhance their understanding and adherence to annotation guidelines:
- Comprehensive Training Programs: Tailored training covers task-specific requirements and includes complex case examples, preparing annotators for various challenges.
- Iterative Feedback Loop: A feedback system provides real-time responses and corrections, fostering continuous improvement and adaptation.
By implementing rigorous quality control measures and maintaining a cycle of continuous training and feedback, Springbord helps businesses achieve reliable and effective AI and ML deployments.
To learn more about how Springbord can enhance your AI and ML deployments through rigorous quality control and continuous training, contact us today!
Challenge: Annotation Tools and Technology Integration
Integrating annotation tools into business systems involves addressing challenges like software compatibility and workflow disruptions, requiring strategic planning and the use of intermediary platforms.
Problem Outline
Integrating the right annotation tools into business systems is fraught with challenges, including software compatibility and workflow disruption. Effective integration requires strategic planning, the use of intermediary platforms, and continuous monitoring to ensure the tools enhance operational efficiency and meet evolving business needs.
Solutions
- Custom Tool Development: Developing bespoke annotation tools tailored to specific business needs enhances operational efficiency. These custom tools are aligned with unique workflows, ensuring seamless integration with existing data systems and providing the flexibility to adapt to evolving business requirements.
- Integration Best Practices: Effective integration of annotation tools into business systems requires a strategic approach:
- Strategic Planning and Phased Implementation: Start with a detailed integration plan that includes phased implementation to manage complexity and minimize disruption.
- Use of Integration Platforms: Integration platforms act as intermediaries, connecting different systems smoothly without extensive coding.
- Continuous Monitoring and Optimization: Continuous monitoring tools detect and resolve integration issues promptly, ensuring optimal system performance.
- Training and Support: Essential training and support help staff utilize new tools effectively within the integrated system
At Springbord, our expertise in system integration ensures that we not only implement the most suitable tools but also optimize your workflows for enhanced productivity and data accuracy. .
Challenge: Data Security and Privacy
Data security and privacy are critical concerns in data annotation, particularly when dealing with sensitive or proprietary information.
Problem Outline
Data security and privacy are important when handling sensitive or proprietary information during the data annotation process. With increasing reliance on AI and machine learning, the risks associated with mishandling training data—such as identity theft or exposure of sensitive information become significant concerns.
Solutions
- Compliance and Standards: Understanding and adhering to international data protection laws like the General Data Protection Regulation (GDPR) and the California Consumer Privacy Act (CCPA) is crucial. These regulations dictate how personal data should be handled and give individuals rights over their data. Ensuring compliance involves adopting lawful bases for data processing and maintaining transparency about data usage.
- Secure Annotation Practices: Implementing stringent security measures is essential to protect data during the annotation process. These practices include:
- Physical Security Measures: Establishing secure facilities with controlled access and surveillance to prevent unauthorized data access.
- Cybersecurity Measures: Utilizing strong encryption for data storage and transfer, implementing role-based access controls, and restricting internet access to minimize risks of data breaches.
- Regular Audits and Compliance Checks: Conducting frequent security audits and ensuring all practices meet or exceed regulatory standards.
At Springbord, our approach involves using advanced security measures and conducting regular reviews and updates to our practices to ensure they align with the latest in data protection regulations.
Challenge: Cost Management
Cost management in data annotation focuses on assessing the financial implications, including cost-effectiveness and ROI, to ensure expenditures align with the anticipated business benefits.
Problem Outline
Managing the costs of data annotation involves evaluating the financial impact, including cost-effectiveness and the return on investment (ROI). This is crucial for businesses to ensure that the expenses associated with data annotation justify the benefits derived from enhanced machine learning models and AI-driven insights.
Solutions
- Cost-Benefit Analysis
Conducting a cost-benefit analysis is essential to determine the financial viability of data annotation projects. This analysis involves calculating the total costs of the project, including direct and indirect expenses, and comparing these costs to the anticipated benefits, such as improved accuracy of AI models and efficiency in operations. The goal is to ensure that the benefits outweigh the costs, which supports the business case for proceeding with the project.
- Outsourcing vs. In-House
Deciding between outsourcing data annotation tasks and maintaining an in-house team involves comparing the costs and benefits of each approach. Outsourcing can be cost-effective, particularly for businesses without the existing infrastructure or expertise to manage large-scale annotation internally. It allows companies to leverage the specialized skills of external vendors, often at a lower cost due to economies of scale. However, maintaining an in-house team may offer benefits such as better control over data security and quality, especially for sensitive or proprietary information.
Springbord helps businesses perform detailed cost-benefit analyses to ensure that their investment in data annotation is justified by measurable returns. Whether you’re considering outsourcing or developing an in-house capability, Springbord can offer insights and services that enhance cost efficiency and project outcomes.
Conclusion
The complexities of data annotation is essential for businesses looking to harness the transformative power of AI and machine learning. By addressing challenges such as scalability, data quality, tool integration, security, and cost management, companies can improve the effectiveness of their AI applications and secure a competitive advantage.
Each of these areas requires careful consideration and strategic planning to ensure that data annotation processes are not only efficient but also aligned with business goals.
Ready to elevate your AI and machine learning projects with expert data annotation services?
Visit Springbord today to discover how our customized solutions can drive your business forward.