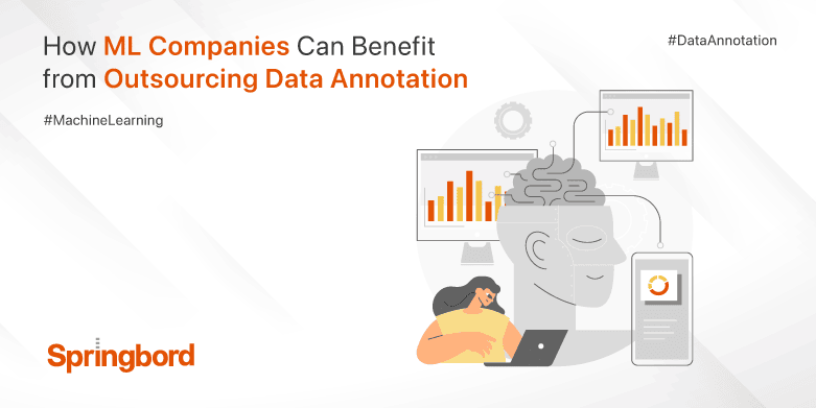
Outsourcing data annotation involves hiring a third-party company to annotate data on behalf of the ML company.
This can save a lot of time and effort for ML companies, as they can focus on their core competencies while the annotation work is done by the outsourcing partner.
Outsourcing data annotation has many benefits, including increased efficiency, cost savings, and improved data quality.
What are the challenges of in-house data annotation?
Data annotation is the process of labeling and categorizing large amounts of data in order to train and improve machine learning algorithms.
While it is a crucial component for many machine learning companies, the process of in-house data annotation can be challenging.
The following are some of the most significant difficulties associated with in-house data annotation and how outsourcing can help alleviate these issues.
- Time-Consuming: In-house data annotation can be an incredibly time-consuming process. Labeling and categorizing large amounts of data can take months or even years to complete. This can slow down the development process and impede the growth of the company.
- Cost: Hiring and training a team of in-house annotators can be expensive. The cost of salaries, training, and resources can add up quickly and significantly impact a company’s bottom line.
- Quality Control: Ensuring the accuracy and consistency of in-house annotated data can be difficult. Inconsistent labeling can lead to biased results and incorrect predictions from the machine learning algorithm.
- Limited Resources: In-house data annotation teams often have limited resources and expertise. This can lead to difficulties in completing complex tasks and implementing new techniques.
How outsourcing data annotation helps machine learning companies
Machine learning (ML) is an exciting field that has the potential to change the world. However, for ML algorithms to work properly, they require vast amounts of high-quality training data. This data must be annotated in order to help the algorithm make accurate predictions. For many ML companies, the process of annotating data can be time-consuming and resource-intensive. This is where outsourcing data annotation comes in.
Data annotation is a crucial aspect of machine learning development, as it provides the necessary data for training and testing machine learning models. It can be a time-consuming and tedious process, but it is essential for the success of any machine learning project. Outsourcing data annotation can help machine learning companies in several ways.
Have quality training data
One of the biggest advantages of outsourcing data annotation is that it gives machine learning companies access to quality training data. Data annotators are trained professionals who have experience in annotating large volumes of data accurately and efficiently. This means that machine learning companies can get their data annotated quickly, without sacrificing quality. With the help of data annotators, machine learning companies can ensure that their data is properly labeled and structured, which is essential for building effective machine learning models.
Define contextual workflows
Data annotation can be a complex process, and it is important to ensure that it is carried out in a consistent and accurate manner. Outsourcing data annotation can help machine learning companies define contextual workflows, which ensure that all data is annotated in the same way, every time. This helps to minimize errors and improve the quality of the training data, which is essential for building effective machine-learning models.
Easily scale for increased the data volume
Machine learning projects can often require large volumes of data, and it can be challenging for machine learning companies to keep up with the pace of data collection. Outsourcing data annotation can help machine learning companies scale their data annotation efforts easily, as they can access a team of trained data annotators who can annotate large volumes of data quickly and efficiently. This helps machine learning companies to keep pace with their data collection efforts and ensure that they have the necessary training data to build effective machine learning models.
Match the speed of the ML project
Machine learning projects can often have tight deadlines, and it can be challenging for machine learning companies to keep up with the pace of data collection. Outsourcing data annotation can help machine learning companies match the speed of their machine learning project, as they can access a team of trained data annotators who can annotate large volumes of data quickly and efficiently. This helps machine learning companies to stay on track with their project timelines and ensures that they have the necessary training data to build effective machine learning models.
Keep checking on bias
Data bias is a major concern in machine learning development, and it is important to ensure that training data is free from bias. Outsourcing data annotation can help machine learning companies keep check on bias, as they can access a team of trained data annotators who are aware of the importance of avoiding data bias. This helps machine learning companies to build models that are fair and unbiased, which is essential for ensuring the success of their machine learning projects.
Provide dynamic security frameworks
Data security is a major concern in machine learning development, and it is important to ensure that training data is protected. Outsourcing data annotation can help machine learning companies provide dynamic security frameworks, as they can access a team of data annotators who are trained in data security best practices. This helps machine learning companies to ensure that their training data is protected and secure, which is essential for building effective machine learning models.
Gives access to proven data annotation expertise
Outsourcing data annotation to a service provider can also give ML companies access to proven data annotation expertise. Service providers have a team of experienced annotators who have the knowledge and skills to annotate data accurately and efficiently. This can help ML companies to save time and resources, and ensure that the annotated data they receive is of the highest quality.
Other ways in which outsourcing data annotation proves advantageous for ML companies
Machine learning (ML) companies rely heavily on data annotation to train their models and make accurate predictions. This process can be time-consuming and resource-intensive, but outsourcing it to a reliable service provider can provide numerous benefits.
Ready compliance to standards
Data annotation is a critical part of the data pre-processing stage, and the annotation process must adhere to strict data privacy and security standards. Outsourcing to a professional service provider ensures that the data annotation is done in compliance with the relevant regulations, such as the General Data Protection Regulation (GDPR) or the Health Insurance Portability and Accountability Act (HIPAA). This reduces the risk of data breaches and enhances the reputation of the ML company.
Consistent and agile solution delivery
Outsourcing data annotation to a professional service provider ensures consistent and agile solution delivery. Service providers have a team of experienced annotators who can handle large volumes of data and provide annotations within tight deadlines. This helps ML companies to focus on their core business and meet the growing demand for their services without being bogged down by the time-consuming data annotation process.
Uninterrupted support
When outsourcing data annotation, ML companies can enjoy uninterrupted support from the service provider. This is particularly important for companies that require constant updates and modifications to their data annotations. Service providers have a dedicated team of support staff who are available 24/7 to ensure that the data annotation process runs smoothly and without any interruptions.
Flexible frameworks
Outsourcing data annotation also provides ML companies with flexible frameworks that can adapt to their specific needs. Service providers have a range of tools and techniques that they can use to provide tailored data annotation solutions that meet the specific requirements of each ML company. This ensures that the data annotation process is streamlined and efficient, providing ML companies with the data they need to make accurate predictions.
Conclusion:
Outsourcing data annotation is an excellent way for ML companies to overcome the challenges associated with collecting and annotating high-quality data.
By partnering with a professional service provider like Springbord, ML companies can access a team of experts who are dedicated to providing high-quality, accurate data annotations.
This can help ML companies improve the accuracy of their models and accelerate the development process.
If you’re looking to outsource data annotation, consider working with Springbord – a leading service provider in the field of data annotation.