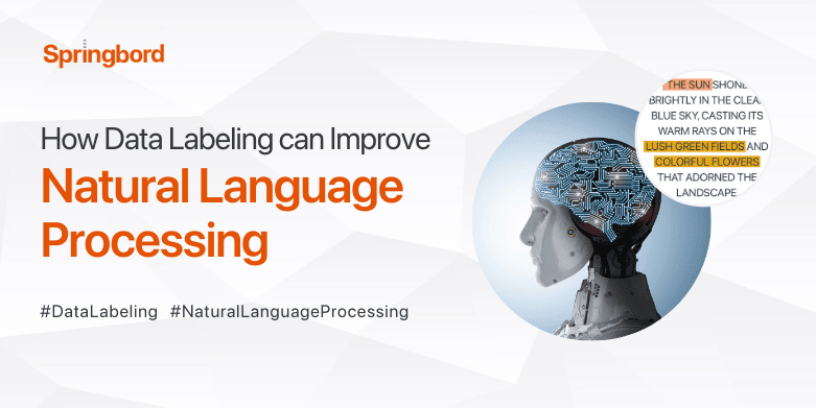
Is improving the precision and dependability of your NLP models a priority? Wondering how data labeling can play a crucial role in achieving these goals? Look no further!
In this article, we will explore the immense potential of data labeling in improving NLP and why it has become a critical aspect for businesses in the B2B sector. Join us on this informative journey as we delve into the world of NLP and uncover the power of high-quality labeled data.
Introduction:
Natural Language Processing (NLP) has revolutionized the way businesses operate in the B2B sector. It encompasses various techniques and algorithms that enable computers to understand, interpret, and generate human language. From sentiment analysis and chatbots to voice recognition and text summarization, NLP has become indispensable in today’s digital landscape.
As the demand for accurate and reliable NLP models continues to grow, organizations face the challenge of acquiring high-quality labeled data. Data labeling, the process of annotating data with meaningful tags or labels, has emerged as a key solution to address this challenge.
In this article, we will explore the importance of data labeling in NLP and how Springbord‘s expertise in data labeling can optimize the performance of NLP models
The Role of Data Labeling in NLP
2.1. Definition and Significance of Data Labeling
Data labeling is the process of annotating or categorizing data to provide meaningful context and structure for machine learning algorithms. In the context of Natural Language Processing (NLP), data labeling involves assigning relevant labels, tags, or annotations to text data, enabling machines to understand and process human language more effectively. Accurate data labeling is crucial for training NLP models, as it helps algorithms identify patterns, extract information, and make accurate predictions.
2.2. Importance of High-Quality Labeled Data for NLP Model Training
High-quality labeled data is the foundation of successful NLP model training. Labeled data enables algorithms to learn the relationship between inputs (text) and outputs (labels or predictions). With accurate labels, NLP models can understand the nuances of language, accurately classify text, extract information, and generate meaningful insights. Without proper data labeling, NLP models may struggle to achieve the desired level of accuracy and reliability.
2.3. Challenges in Data Labeling for NLP
Data labeling for NLP poses specific challenges due to the complexity and variability of human language. Some common challenges include:
a. Subjectivity and Ambiguity: Language often contains subjective or ambiguous elements that require human judgment to assign appropriate labels. For instance, understanding sentiment or identifying sarcasm can be challenging for algorithms without human context.
b. Language Diversity: NLP models need to handle diverse languages, dialects, and regional variations. Data labeling should consider these linguistic nuances to build models that are robust and adaptable across different contexts.
c. Scalability: As the amount of available data grows exponentially, manual data labeling becomes time-consuming and expensive. Scaling up data labeling efforts without compromising quality can be a significant challenge.
d. Domain Expertise: Different industries and domains have unique terminologies, jargon, and linguistic nuances. Without access to domain experts, data labeling may lack accuracy and industry-specific insights.
2.4. Benefits of Outsourcing Data Labeling Tasks
Outsourcing data labeling tasks offers several advantages for businesses looking to improve NLP performance:
a. Access to Domain Experts and Linguistic Resources: By partnering with a data labeling service provider, businesses gain access to professionals with expertise in various domains. These experts understand the specific language and context, ensuring accurate and industry-specific data labeling.
b. Scalability and Flexibility: Outsourcing allows businesses to scale their data labeling efforts based on their requirements. Service providers can handle large volumes of data efficiently, ensuring timely delivery and scalability without compromising quality.
c. Cost-Effectiveness and Time Efficiency: Outsourcing data labeling eliminates the need to invest in infrastructure, tools, and human resources required for in-house labeling. It also saves valuable time, allowing businesses to focus on core competencies while leveraging the expertise of data labeling specialists.
d. Quality Assurance and Consistency: Data labeling service providers follow standardized annotation guidelines, ensuring consistency and quality in labeled data. They employ quality control measures and validation processes to maintain high accuracy and reliability.
Optimizing NLP Performance through Data Labeling
3.1. Supervised Learning and the Role of Labeled Data
Supervised learning is a popular approach in NLP that relies on labeled data to train algorithms. Labeled data provides explicit examples of inputs and corresponding outputs, allowing models to learn patterns and make accurate predictions. The availability of high-quality labeled data is crucial for training NLP models using supervised learning algorithms effectively.
3.2. Training Data Selection and Annotation Guidelines
Effective data labeling involves the careful selection of training data and the development of comprehensive annotation guidelines. Training data should be diverse, and representative, and cover a wide range of language patterns and use cases. Annotation guidelines provide instructions and standards for labeling data consistently, minimizing labeling errors, and ensuring the desired model performance.
3.3. Active Learning Techniques to Reduce Labeling Efforts
Active learning techniques help reduce the amount of labeled data required by selecting the most informative samples for annotation. Algorithms can prioritize uncertain or challenging examples, seeking human input only for data points where models exhibit low confidence. This approach optimizes the data labeling process by focusing efforts on samples that contribute the most to improving model performance.
3.4. Impact of High-Quality Labeled Data on NLP Accuracy and Reliability
High-quality labeled data significantly impacts the accuracy and reliability of NLP models. Proper data labeling enhances the models’ ability to understand context, detect sentiment, extract key information, and perform other language-related tasks. With accurate labeling, NLP models can provide more accurate insights, improve customer interactions, automate business processes, and enhance decision-making.
Best Practices for Outsourcing Data Labeling
Data labeling plays a pivotal role in improving Natural Language Processing (NLP) capabilities, enabling businesses to extract meaningful insights and enhance their operations. To fully harness the benefits of data labeling, it is crucial to adopt best practices when outsourcing this task. Here are four key practices for businesses seeking to leverage data labeling services effectively.
4.1 Identifying Reliable Data Labeling Service Providers
When outsourcing data labeling, partnering with a reliable service provider is paramount. Look for providers with a proven track record in NLP-related projects and extensive experience in data annotation. Evaluate their expertise in handling various NLP tasks such as sentiment analysis, entity recognition, and intent classification. Verify their ability to deliver accurate and high-quality labeled data, which is essential for training robust NLP models.
Choosing a service provider with a strong reputation and positive client testimonials can instill confidence in the quality of their work. Additionally, considering the provider’s scalability, flexibility, and ability to meet project deadlines will ensure a smooth and efficient data labeling process.
4.2 Developing Clear Annotation Guidelines and Task Specifications
To obtain accurate and consistent labeled data, clear annotation guidelines and task specifications are crucial. Clearly define the objectives of the NLP project and communicate them effectively to the data labeling service provider. Establishing comprehensive annotation guidelines that cover specific labeling conventions, domain-specific terms, and potential challenges ensures consistency across the labeled data.
Collaborate closely with the service provider during the initial stages to create a shared understanding of the annotation process. Encourage ongoing feedback and clarification to address any ambiguities or uncertainties. This iterative approach will lead to refined guidelines and improved data quality over time.
4.3 Ensuring Data Privacy and Security
Maintaining data privacy and security is of utmost importance when outsourcing data labeling tasks. The service provider should have robust data protection measures in place, including secure data transfer protocols, restricted access to sensitive information, and comprehensive confidentiality agreements. Compliance with data protection regulations, such as the General Data Protection Regulation (GDPR), is crucial, especially when handling personally identifiable information (PII).
Before engaging a data labeling service provider, thoroughly evaluate their security practices and policies. Ensure that they have implemented stringent measures to safeguard your data and protect the privacy of your customers and business.
4.4 Establishing Effective Communication Channels
Effective communication between the business and the data labeling service provider is vital for successful collaboration. Establish clear lines of communication, ensuring that both parties can easily address questions, provide clarifications, and resolve issues promptly.
Regular communication helps maintain alignment regarding project goals, timelines, and any changes or updates required during the annotation process. By fostering open and transparent communication, businesses can ensure that the labeled data meets their specific NLP requirements.
Overcoming Challenges and Mitigating Risks
While outsourcing data labeling offers numerous benefits, it also presents potential challenges and risks. Adopting appropriate strategies for risk mitigation and quality control is crucial for ensuring the success of NLP projects.
5.1 Potential Challenges in Outsourcing Data Labeling
Outsourcing data labeling may introduce challenges such as linguistic nuances, domain expertise, and contextual understanding. These challenges can impact the accuracy and quality of the labeled data. Language-specific subtleties, dialects, or jargon require careful attention during the annotation process to avoid misinterpretations.
In addition, the service provider may lack a deep understanding of the specific industry or domain, potentially leading to suboptimal annotations. Overcoming these challenges requires close collaboration, feedback loops, and continuous improvement to align the labeled data with the desired NLP outcomes.
5.2 Strategies for Risk Mitigation and Quality Control
To mitigate risks associated with outsourced data labeling, implementing robust quality control measures is essential. Employing a multi-tiered annotation process that includes quality checks, inter-annotator agreement, and regular audits can help ensure consistent and reliable labeled data.
Implementing automated or manual spot checks, where a subset of annotated data is independently reviewed for accuracy, helps maintain quality standards. Continuous feedback loops between the business and the service provider enable timely adjustments and improvements throughout the annotation process.
5.3 Monitoring and Evaluation of Outsourcing Partnerships
Continuous monitoring and evaluation of the outsourcing partnership are critical to track progress, identify areas for improvement, and measure the effectiveness of the labeled data in enhancing NLP models. Regularly assess the quality of the labeled data and the performance of NLP models trained on this data. Compare the results against predefined benchmarks and evaluate the impact on business operations and decision-making.
Gaining insights from monitoring and evaluation allows businesses to make informed decisions about optimizing their outsourcing partnerships and fine-tuning their NLP strategies.
Conclusion:
In conclusion, data labeling plays a pivotal role in improving Natural Language Processing (NLP) and empowering businesses in the B2B sector. By outsourcing data labeling tasks, companies can unlock the potential for enhanced business efficiency and gain a competitive advantage.
The future of data labeling and NLP in B2B holds great promise, as advancements in technology continue to push the boundaries of what is possible. Don’t miss out on this transformative opportunity!
Take the first step towards improving your NLP models by embracing the power of high-quality labeled data. Contact us today to explore how Springbord, a leading data labeling service provider, can help revolutionize your NLP applications.