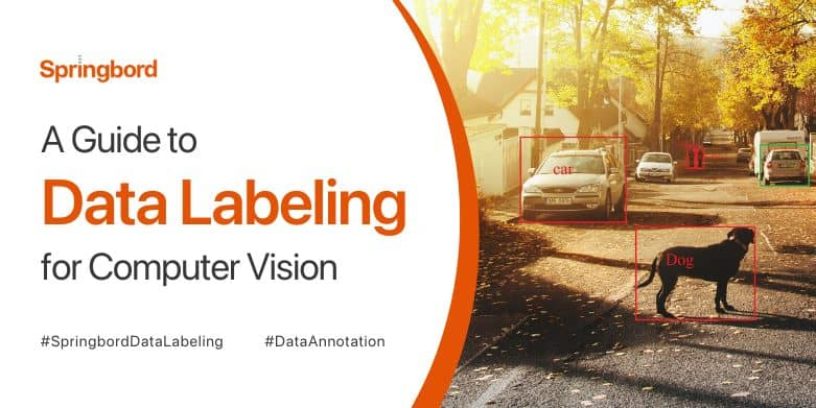
Data labeling for computer vision is an essential task in the world of artificial intelligence and machine learning. It involves the process of marking images, videos, and other data types with specific labels or annotations to help computers recognize and classify them accurately.
This process is critical in developing computer vision models that can be used in various industries such as healthcare, transportation, and e-commerce.
In this guide, we will explain what data labeling is, its importance in computer vision, and how we can help you with your data labeling needs.
What is Data Labeling for Computer Vision?
Data labeling is the process of adding descriptive metadata to images or videos to help machines learn to recognize patterns and objects.
In the context of computer vision, data labeling is essential for training machine learning algorithms to perform tasks such as image recognition, object detection, and semantic segmentation.
Why is Data Labeling Important?
Accurate and consistent data labeling is critical for the success of machine learning algorithms. Without high-quality labeled data, these algorithms cannot learn to recognize patterns and make accurate predictions.
This is especially important in applications such as autonomous vehicles, medical imaging, and surveillance, where the consequences of inaccurate predictions can be severe.
How Does Data Labeling Work?
Data labeling for computer vision typically involves three steps: data collection, data labeling, and data validation. The data collection step involves gathering images or videos that will be used to train the machine learning algorithm.
The data labeling step involves adding descriptive metadata to each image or video, such as object labels, bounding boxes, or semantic segmentation masks.
The data validation step involves checking the accuracy and consistency of the labeled data to ensure that it meets the required standards.
Challenges in Data Labeling for Computer Vision
Data labeling for computer vision can be difficult and time-consuming, and there are a number of obstacles that must be overcome to produce reliable results. The most frequently encountered difficulties include:
- Ambiguity: Images or videos can be ambiguous, making it difficult to accurately label objects or patterns.
- Diversity: Images or videos can vary widely in terms of lighting, camera angle, and resolution, making it challenging to develop consistent labeling standards.
- Scalability: Data labeling can be a time-consuming process, especially for large datasets.
At Springbord, we have developed strategies to overcome these challenges, including using advanced labeling tools and techniques, developing comprehensive labeling guidelines, and leveraging our team’s experience to ensure high-quality results.
How Can Springbord Help with Data Labeling for Computer Vision?
At Springbord, we offer comprehensive data labeling services to help businesses leverage computer vision technology.
We use state-of-the-art tools and techniques to ensure accurate and reliable data labeling, and we work with you to customize our services to meet your specific needs.
Our team of experienced data labelers has a deep understanding of computer vision and can label your data accurately and efficiently. We use a combination of manual and automated labeling techniques to ensure the highest quality results, and we work closely with you to ensure that your data is labeled to your exact specifications.
Conclusion
Data labeling is a crucial aspect of computer vision, and it is essential to have accurate and reliable data to train your models.
At Springbord, we offer comprehensive data labeling services to help businesses leverage this technology and gain a competitive advantage.
Our experienced team of data labelers uses state-of-the-art tools and techniques to ensure accurate and efficient labeling, and we work closely with you to customize our services to meet your specific needs.
Contact us today to learn more about how we can help with your data labeling needs.