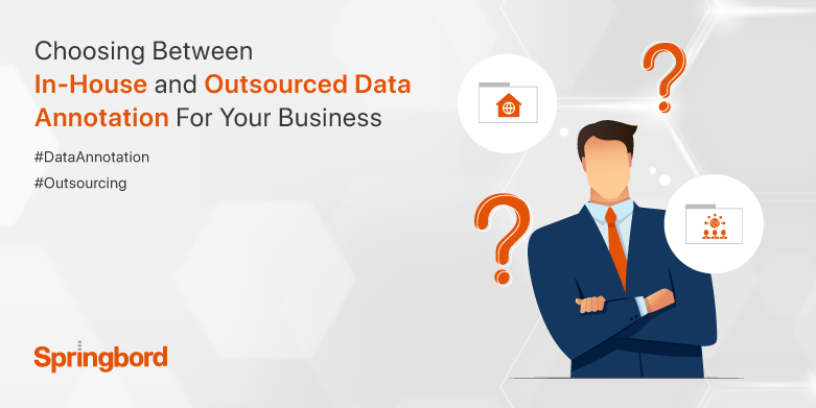
Annotating data means examining data samples for relevance and adding descriptive labels. Images, videos, audio files, and written text are all examples of data. Put another way, a data label or tag is only a descriptive indicator of the nature of the data it accompanies. The foundation of any artificial intelligence or machine learning model must begin here. The model can learn more from the data if it is labeled.
Due to the fact that AI models need a sizable amount of annotated data before being deployed, many businesses planning to build machine learning algorithms will have to make a decision early on. Whether to build a team in-house, resort to crowdsourcing, or hire a reputable outsourcing company. We take a look at the differences between the two.
In-house Data Annotation
Some people believe that having your own in-house data labeling team is preferable since it allows for closer monitoring, higher levels of security, and greater IP protection. However, generating the training data required to construct AI models can be costly, complex, and time-consuming. Unfortunately, few businesses can afford to hire, train, and maintain a team of data labelers. Costs might quickly escalate when you factor in things like renting larger offices and creating specialized software and equipment. The other issue is that data labeling is typically done on a project-by-project basis, so there will be a lot of personnel turnover. This necessitates starting the hiring and training process from scratch for every new endeavor.
Data annotation is crucial, but adding it to your engineers’ to-do lists is unwise when so much depends on it. A significant amount of time may be taken up with labeling massive amounts of data, or worse, this crucial task may be neglected. Even with in-house data annotation tools, it may be impossible to label massive amounts of data in time for a project’s deadline or to respond quickly enough to requests to add new data or labels to a machine learning training data set.
Outsourcing Data Annotation
Many organizations find that partnering with an external, professional, data-annotation firm offers the “best of both worlds.” Companies can save money without compromising quality by teaming up with a well-established and trustworthy partner. These experts work for data labeling firms that hire qualified, professional annotators who are flexible enough to meet any need and experienced enough to use the latest and greatest in annotation technology. If you plan on returning with additional data sets over time, outsourcing can help establish a long-term connection with your partner. Your outsourcing partner can easily reassign some of their workers to your account if you expect a seasonal rise and need to scale up the workforce. This eliminates the time and effort required for recruiting, employing, training, and ultimately letting go of employees as demand for their services declines.
The expertise of an outside company that specializes in data annotation is invaluable. No team member has to juggle competing priorities to meet a client’s product launch deadline or develop a new system. Project managers at a data annotation company ensure the job gets done correctly, is safe, and is finished on schedule.
The Advantages of Outsourcing Data Annotation
Freedom to focus on core developmental work
Preparing the data sets is a difficult but essential first step in training ML models. Assigning data scientists to activities as mundane as cleaning and classifying the data diverts their efforts away from more productive endeavors. Consequently, problems would arise in the development cycle as a result of overlaps in processes that could cause delays.
The entire system is simplified and developed in real-time when the process is outsourced. When you outsource your data, your staff can concentrate on what they do best: develop robust AI-based solutions.
Safety And Reliability Guarantees
With a team of skilled data labelers working solely on your project, you can rest assured that it will be completed on time and to your satisfaction. By drawing on their prior experience with various data sets and developing their data labeling skills, these professionals improved data labeling for ML and AI applications.
Capacity To Manage Massive Amounts Of Data
Data labeling is labor-intensive, and thousands of data sets need to be appropriately labeled and annotated for a typical AI project. Nonetheless, the quantity of data involved is highly project-specific, so a rise in demand may lengthen the due dates for your in-house teams’ milestones. When the volume of data increases, you may need to bring in help from other teams, which could lower productivity.
Conclusion
Data labeling is labor-intensive, and thousands of data sets need to be appropriately labeled and annotated for a typical AI project. However, the quantity of data involved differs widely depending on the nature of the project, and this surge in demand may lengthen the timelines for your internal teams’ deliverables. As the volume of data grows, you may need to recruit help from other departments, which could lower productivity.
At Springbord, we are always here to help and have the experience and knowledge to deal with fluctuating data loads. Additionally, we have the capability and resources to scale with your project as it grows.