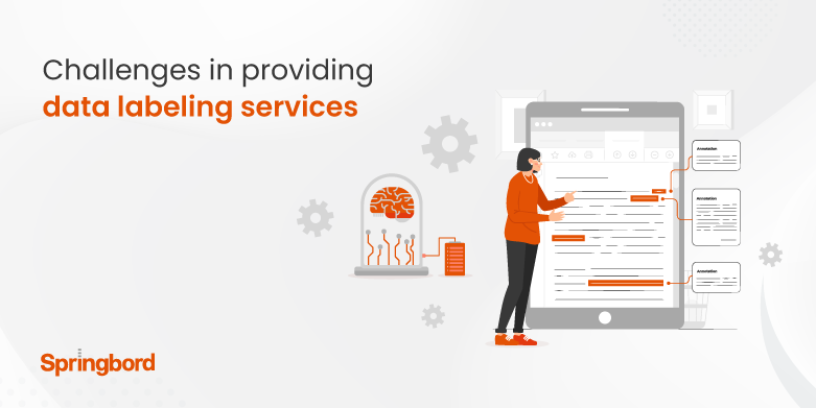
Both automation and human factors play a crucial role in the success of any data labeling or annotation projects. The groundwork involved in building these projects is time-consuming, complex and expensive. To a large extent, the success of any such projects depends on data scientists, data engineers and data modelers. In fact they are the ones who identify data for aggregation, cleansing, augmentation and labeling. This impacts the outcome of any algorithm or machine learning tasks. The major factors that pose a challenge to any data labeling services are:
Lack of subject matter experts: A data analyst gets insights from data, but they also have to be domain experts when it comes to data labeling. Many organisations assume this role to be a clerical one and often end of hiring resources without any background in the subject. Today, this is the foremost challenge facing most companies in the industry: lack of expert resources in a data labeling domain. Any data modeling effort that is not based on insight is bound to add to the cost and level of complexity.
Improper tools: One of the first things that businesses should understand is that different types of data require different tools. For data companies that build in-house tools, it is important to build tools consistent with the data requirements and complexity. However, most use their proprietary tool for a variety of data labeling tasks, which only increases the operational data risk. In other words, the existing in-house annotation tools may not support a client’s different business scenarios.
Lack of a secured environment: Now that data security standards have become global, companies are often found not complying with regulatory requirements. Due to the high turnover of roles in the industry and increased competition, companies often hire resources without proper validation and checks. In addition, there is no proper training given to the staff on the global security standards. Resources are often given a brief introduction of their roles and expectation, and are tasked with meeting the delivery schedule. It is also a norm for companies to subcontract the tasks to other companies, thereby increasing the risk to data.
Inadequate quality metrics: Ensuring the quality of data is often the victim in large-scale data annotation or labeling projects. With the growth of AI-driven processes, data that is not primed for usage is fed into the system, which is then scaled up for handling more data sources. Companies tend to overlook the fact that it is the data that determines the success of any AI implementation. Data is not considered or treated according to its peculiarity, but is often subjected to technology and generic bias. The upshot of such an action is that the entire exercise becomes ineffective right from the beginning itself.
Data labeling is an evolving sector, which is still human-driven with an AI-based system. There is an existential risk when companies do not use personalized annotation tools and services according to customer requirements. It is more complex than one would like to think. The cost of not complying to the data labeling needs –insight, techniques, security and quality—is often very high, and companies should bear these in mind when handling data labeling projects.