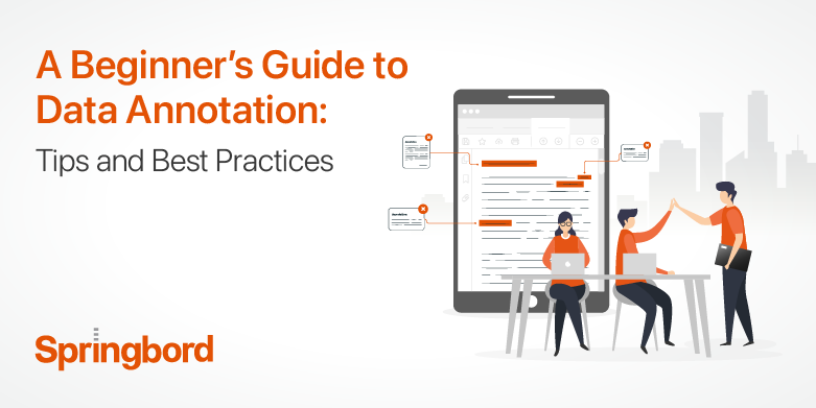
As we get into the digital age, the ability to effectively use artificial intelligence (AI) and machine learning (ML) hinges significantly on the quality of data these technologies are fed. Data annotation is the process that ensures this quality by labelling data in ways that are meaningful for machines to process and learn from.
This blog explores the essential role of data annotation in transforming raw data into a structured resource for machine-driven analysis and decision-making, aiming to enhance business operations across various sectors.
Overview of Data Annotation
Data annotation is a crucial process where data in various formats (like text, images, video, or audio) is labeled or tagged with relevant information to make it interpretable by machine learning algorithms.
This process is essential for training AI models to recognize and understand the content and context of the data they process.
Significance in Business Context:
In AI and ML, data annotation acts as the bridge between human expertise and machine learning efficiency. It allows businesses to convert unstructured data into rich, actionable insights, driving more informed decisions.
Whether it’s improving product categorization in e-commerce, enhancing customer interaction through chatbots, or optimizing logistics through smarter route planning, the implications are vast and varied.
Importance for Business Owners
For business owners, data annotation is fundamentally important as it directly impacts the efficiency and effectiveness of machine learning projects which are crucial for maintaining competitive advantage.
Here’s how:
- Enhanced Product Development: By annotating data, businesses can train models to better understand consumer behaviours and preferences, leading to improved product designs and features that are closely aligned with market demands.
- Improved Customer Experiences: Data annotation allows AI systems to provide personalized experiences, predict customer needs, and offer timely solutions, thereby enhancing customer satisfaction and loyalty.
- Operational Efficiencies: Annotated data supports the automation of routine tasks, predictive maintenance, and optimized supply chains, which can significantly reduce operational costs and improve service delivery.
- Informed Decision-Making: With accurate data annotation, businesses gain deeper insights from their data, enabling better strategic decisions based on trends and analytics rather than assumptions.
Contact Springbord for tailored solutions to ensure that your data-driven projects are built on accurately annotated data, enhancing both precision and efficiency.
Types of Data Annotation
Data annotation is a fundamental process in preparing data for machine learning and AI applications, and it comes in several forms, each suited to specific types of data and applications.
Text Annotation
Text annotation is crucial for natural language processing (NLP) applications such as sentiment analysis, chatbot training, and customer feedback analysis. By labelling text data, machines can learn to interpret human language, identify sentiments, categorise content, and recognise named entities like people, places, and organizations.
This process not only enhances the relevance and efficiency of search engines and recommendation systems but also supports complex document processing tasks in legal, financial, and customer service domains.
Image/Video Annotation
Image and video annotation are integral to computer vision, enabling machines to recognize and interpret visual data. This type of annotation is used extensively in surveillance systems, quality control processes in manufacturing, and retail analytics, where it helps in object detection, facial recognition, and behaviour analysis.
Through techniques like bounding boxes, polygonal annotations, and landmarking, annotated images and videos train AI models to accurately identify and react to visual cues in their environment.
Audio Annotation
Audio annotation involves transcribing and tagging audio recordings to train voice-assistant technologies and improve customer service interactions through speech recognition systems.
By annotating audio data, AI models can understand spoken language, detect emotional nuances, and respond appropriately in interactions. This type of annotation is particularly beneficial for developing more responsive and understanding AI-driven support systems in various service industries.
At Springbord, we leverage our expertise in these areas to provide businesses with precise and efficient data annotation solutions. Our skilled teams ensure the highest quality of data annotation, tailored to meet the specific needs of your AI and ML projects, driving better accuracy and performance in your applications.
Choosing the Right Annotation Tools and Technology
Selecting the optimal tools and technology for data annotation is a critical decision that can significantly affect the efficiency and effectiveness of your business’s AI and machine learning initiatives.
Here’s a breakdown of the leading tools and technologies, along with insights into proprietary versus open-source options and tips for integration.
Overview of Leading Data Annotation Tools and Technologies
For business applications that require high precision and scalability, several annotation tools stand out:
- Labelbox: Known for its customization capabilities, Labelbox allows you to tailor the annotation environment to your specific needs, which is particularly beneficial for unique or complex projects.
- CVAT: This tool is excellent for video and image annotation, offering features like frame-by-frame annotation and varied annotation types like polygonal segmentation.
- Kili: Kili shines with its collaborative environment, allowing multiple annotators to work simultaneously, thus speeding up the annotation process.
- Labelstudio: As a versatile open-source tool, Labelstudio supports a wide range of data types and offers customizable workflows, making it a strong choice for projects requiring specific adaptations.
Proprietary vs. Open-Source Annotation Tools
Proprietary Tools:
- Pros: Often come with comprehensive support and maintenance, more controlled updates, and generally include detailed documentation and customer service.
- Cons: Can be expensive, especially at scale, and may lock you into specific formats or workflows.
Open-Source Tools:
- Pros: Typically free to use, highly customizable, and have the advantage of community-driven improvements and support.
- Cons: Might require more technical skill to implement and maintain, and support can be inconsistent, depending on the community’s activity.
Integration with Existing Business Systems
To ensure that data annotation tools seamlessly integrate with your existing systems, consider the following tips:
- API Availability: Tools like Kili and Labelbox offer robust APIs that facilitate integration with existing data management systems and ML pipelines.
- Compatibility: Ensure the tool is compatible with the data formats your business commonly uses and can scale with your data needs.
- Security: Choose tools that comply with your industry’s data security standards. For example, Cogito adheres to GDPR, CCPA, HIPAA, and SOC 2 Type II certifications, making it suitable for sensitive data handling.
For businesses like Springbord, which prioritize efficiency and data security, selecting the right data annotation tools is crucial.
Springbord’s expertise in data management can help businesses integrate these tools effectively, ensuring that data annotation processes are optimized to enhance AI and ML project outcomes.
Best Practices for Effective Data Annotation
Data annotation plays a crucial role in the development of machine learning models by providing the necessary training data to improve accuracy and functionality.
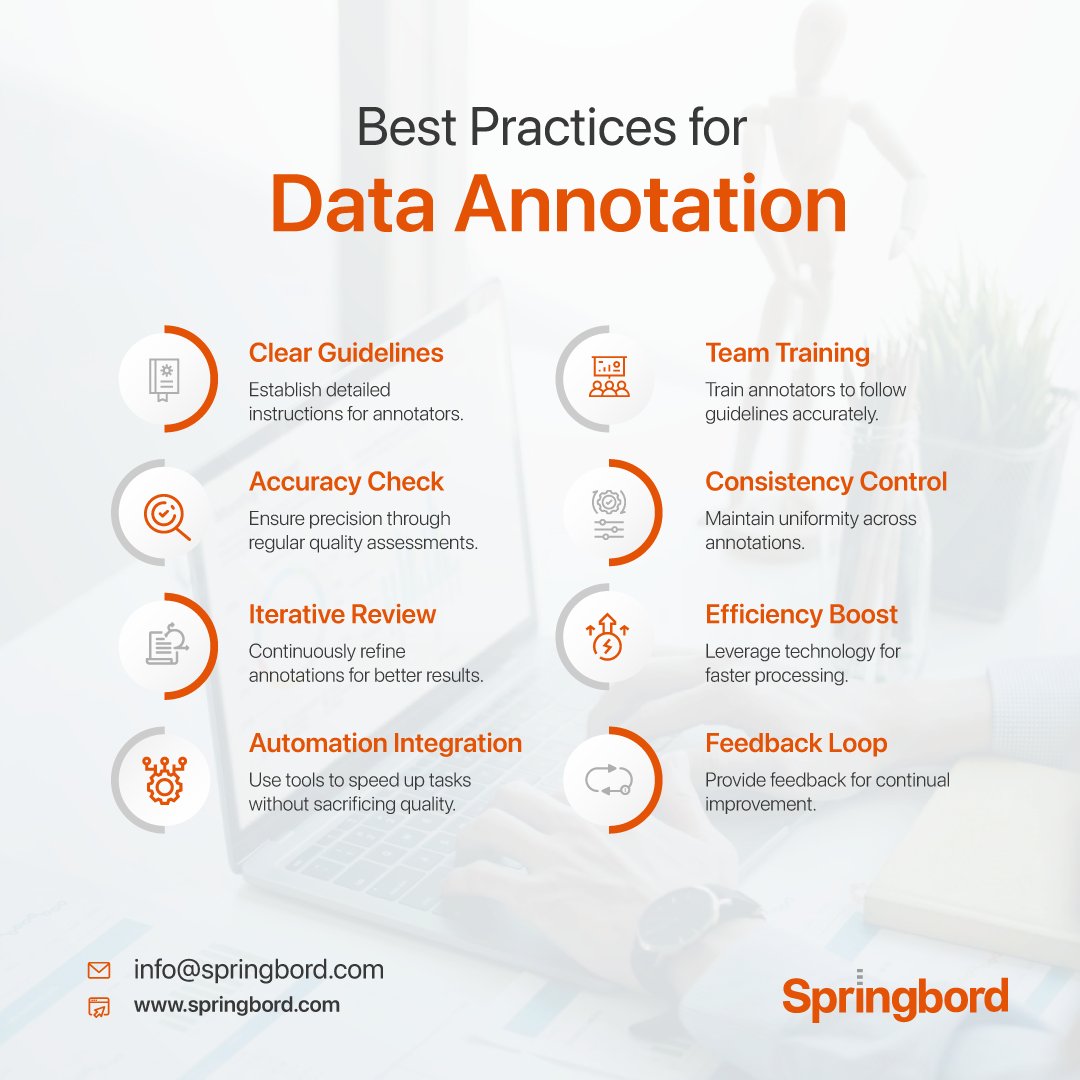
For businesses leveraging AI, particularly in high-stakes sectors such as automotive, financial services, and healthcare, robust annotation practices are not just beneficial but essential.
Setting Up the Annotation Process
Setting up the annotation process is a foundational step in preparing data for machine learning and AI applications.
It includes choosing the right tools and technologies that facilitate the annotation tasks and organizing a team of annotators who are trained to follow the guidelines precisely.
- Defining Annotation Guidelines
The first step in a successful data annotation project is to establish clear and comprehensive guidelines. These guidelines must specify the attributes of interest, the format of the annotations, and the standards for quality and accuracy. This clarity helps ensure consistency across different annotators and reduces ambiguity in the data processing stages.
Springbord excels in customizing these guidelines to meet the unique needs of various industries, ensuring that the annotated data aligns perfectly with client objectives.
- Ensuring Accuracy and Consistency
Accuracy and consistency in data annotation are achieved through a combination of skilled human annotators and rigorous quality control procedures. Regular training sessions, detailed feedback loops, and a system of checks and balances are integral to maintaining high standards.
Springbord leverages both human expertise and technological tools to guarantee that data annotation meets the highest quality standards, with a focus on minimizing errors and biases.
Importance of Iterative Review and Quality Control Processes
Iterative reviews are critical in the annotation process. By regularly evaluating the annotated data, it’s possible to identify and correct errors early in the project lifecycle. This continual refinement helps in fine-tuning the models’ performance over time.
Springbord implements comprehensive quality control measures that include random sample checks and inter-annotator agreement metrics to ensure data reliability and validity.
Leveraging Automated Tools for Efficiency
Automated tools and AI-assisted technologies can significantly enhance the efficiency and accuracy of data annotation.
Tools like machine learning algorithms for pre-annotation, active learning models that prioritize difficult or uncertain samples, and automated validation systems can reduce the workload on human annotators and speed up the annotation process without compromising on quality.
Springbord incorporates state-of-the-art technology to streamline the annotation process, enabling faster turnaround times and cost efficiency for businesses.
Conclusion
Data annotation is essential for leveraging AI and machine learning effectively, transforming raw data into strategic assets that enhance decision-making and operational efficiency across various industries. As the digital landscape evolves, the importance of high-quality data annotation cannot be overstated.
Springbord offers expert data annotation services tailored to meet specific industry needs, ensuring accurate, efficient, and scalable solutions.
Ready to transform your data into a powerful tool for innovation and growth?
Discover how Springbord’s specialized data annotation services can enhance your AI and ML projects.
Visit our website to learn more about our services and how we can help you achieve precision and efficiency in your data-driven initiatives.