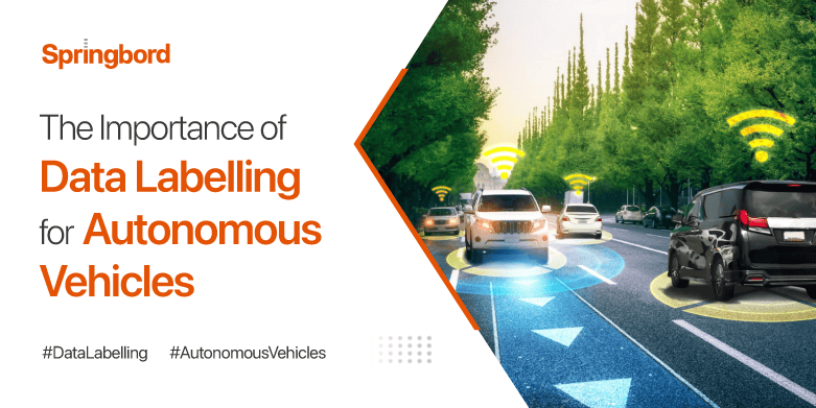
In the ever-evolving landscape of autonomous vehicles, a crucial process forms the bedrock of their capabilities – accurate data labelling. This process is pivotal in empowering these vehicles to not only understand their environment but also navigate it with precision.
In essence, data labelling involves annotating vast datasets, including images and sensor readings, to provide autonomous systems with a comprehensive grasp of their surroundings.
This blog delves deep into the profound importance of data labelling for autonomous vehicles, shedding light on its pivotal role in steering the trajectory of the transportation industry’s future.
Empowering Object Recognition and Classification
The essence of autonomous vehicles’ capabilities resides in supervised learning, with data labelling as its linchpin.
Through meticulously assigned labels, autonomous systems learn to differentiate between pedestrians, vehicles, road signs, and other crucial entities, forming the basis of their ability to comprehend their surroundings.
Nurturing Object Detection Systems
Object detection stands as the lifeblood of autonomous driving, enabling vehicles to identify and track various objects in real-time.
The precision in object placement and attribute annotations provided by data labelling is instrumental in training models to accurately perceive and respond to their environment.
Elevating Semantic Segmentation
Semantic segmentation is a process of dissecting scenes into distinct regions and assigning labels to each pixel.
Data labelling plays a pivotal role in elevating this process, allowing autonomous vehicles to grasp the spatial distribution of objects. This, in turn, enhances their contextual awareness and decision-making capabilities.
Enhancing Sensor Fusion
The symphony of autonomous vehicles’ perception emerges from the fusion of data from various sensors like cameras, LiDAR, and radar.
The integration of this sensor data into a cohesive understanding of the surroundings is facilitated by precisely labelled and time-synced data, which in turn, sharpens the vehicle’s perception and informed decision-making.
Ensuring Safety and Reliability Through Precision Labelling
Mitigating the risk of false positives and negatives is a critical component of data labelling.
Accurate categorization of training data is imperative to minimize the chances of accidents and erroneous decisions by autonomous vehicles, ultimately bolstering road safety.
Handling Complex Scenarios
Data labelling introduces diverse and intricate driving scenarios into the training data.
This equips autonomous vehicles to proficiently navigate real-world situations, enhancing the overall reliability of their performance.
Validating Autonomous Systems
The accuracy of data labelling is paramount when it comes to evaluating and validating the effectiveness of autonomous systems.
By comparing the results to the meticulously labelled ground truth, developers can gauge the system’s capabilities, make necessary refinements, and foster a higher level of trustworthiness.
Compliance with Regulatory Standards
Data labelling is instrumental in meeting the stringent regulatory standards and safety requirements governing autonomous vehicles.
Properly labelled data showcases a systematic approach to testing and validation, instilling confidence both in regulatory bodies and the general public.
Navigating Techniques and Technologies in Data Labelling
The process of data labelling for autonomous vehicles is not static; it’s dynamic and ever evolving.
This involves the utilization of various techniques and technologies to meet the demands of this cutting-edge field.
Manual Vs Machine Learning Algorithms
Human In the data labelling process, manual data labelling offers high precision but comes with drawbacks due to its time-consuming and expensive reliance on human labor. On the other hand, machine learning algorithms, such as semi-supervised learning, efficiently lessen the manual effort needed for data labelling, while active learning enhances model performance by smartly selecting informative samples for annotation.
Confronting Challenges and Ethical Considerations
While data labelling undoubtedly fuels the development of autonomous vehicles, it is not without its challenges. The complex and diverse scenarios that vehicles encounter demand comprehensive data labelling. Moreover, the need to continuously update data to match the ever-changing driving conditions presents a significant challenge. A potential solution lies in simulation-based augmentation, bridging the gap between real-world and simulated data.
Additionally, data labelling must grapple with bias and ethical concerns. Biases can inadvertently seep into AI systems through both human annotators and training data imbalances. Striking a balance between technological progress and ethical integrity is a shared responsibility, involving researchers, governments, and industry stakeholders.
The Significance of High-Quality Data Labelling
At the crux of it all, the quality of data labelling profoundly impacts the performance and success of autonomous vehicles. The accuracy and reliability of AI models are inherently linked to the quality of the labelled data. Precise labelling empowers vehicles to make well-informed decisions, bolstering safety, and nurturing trust among the public and regulatory bodies alike.
The collaborative efforts between industry leaders and data service providers, such as Springbord, equip businesses to navigate the dynamic landscape of autonomous transportation. Springbord’s specialized data solutions aptly highlight the paramount importance of precise data labelling in revolutionizing the world of self-driving vehicles.
Conclusion:
In the rapidly advancing world of autonomous vehicles, data labelling emerges as an invaluable cornerstone. This pivotal process, by meticulously annotating varied elements – from pedestrians to road signs, equips these vehicles to traverse diverse landscapes with unparalleled safety and reliability. Each annotation serves as a lesson, fine-tuning their proficiency to handle intricate real-world challenges.
Yet, the path isn’t without hurdles. As innovative solutions are deployed to expedite data labelling, ethical and bias-related concerns loom large. These issues underscore the need for a delicate balance between technological advancements and ethical responsibility—a mantle shared by researchers, industry experts, and policymakers.
Companies like Springbord, which provide data labelling services, are at the forefront of high-quality data solutions and are instrumental in this evolution. Their endeavors emphasize the critical role of data labelling in the autonomous transportation landscape. Through their expertise, it becomes evident that the safety, efficacy, and public trust in self-driving vehicles are deeply rooted in the precision of data labelling. As we navigate towards a future dominated by these vehicles, the understanding becomes clear: their success hinges on the accuracy and quality of this foundational process. This insight underscores the paramount importance of data labelling, with companies like Springbord leading the charge, as we accelerate into an autonomous-driven era.