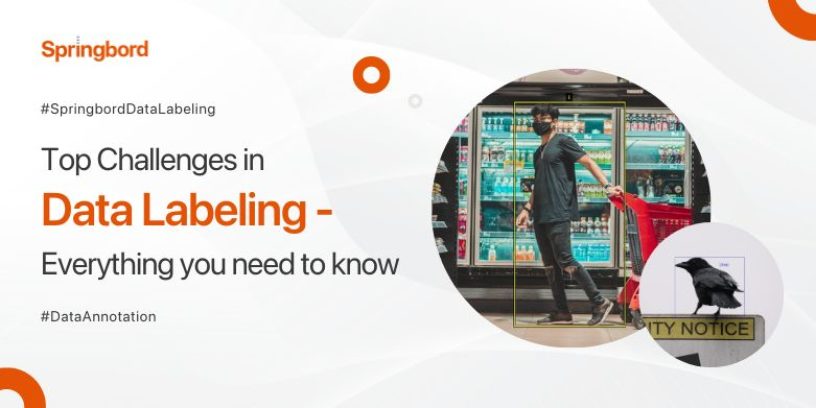
In today’s digital age, data is the backbone of many businesses. And with machine learning becoming increasingly popular, the importance of data labeling cannot be overstated. However, data labeling is a complex process that involves significant challenges. From ensuring the accuracy of the labels to managing large volumes of data, data labeling can be a time-consuming and resource-intensive task.
At Springbord, we understand these challenges and have developed strategies to help our clients overcome them. In this article, we’ll take a closer look at the top challenges in data labeling and provide you with the insights you need to succeed.
Challenge 1: Ensuring Accuracy
One of the biggest challenges in data labeling is ensuring accuracy. The quality of the labeled data directly impacts the performance of the machine-learning model. A single incorrect label can have a significant impact on the accuracy of the model. To ensure accuracy, it’s important to have a thorough labeling process that includes multiple rounds of review and validation.
At Springbord, we use a combination of automated and human labeling to ensure accuracy. Our labeling experts have years of experience in the field and are trained to identify and correct labeling errors. We also have a rigorous quality assurance process in place to ensure the accuracy of the labeled data.
Challenge 2: Managing Large Volumes of Data
Another major challenge in data labeling is managing large volumes of data. As the amount of data grows, so do the time and resources required to label it. This can make it difficult to meet tight deadlines and can also impact the quality of the labels.
At Springbord, we use a combination of technology and human expertise to manage large volumes of data. We use automated tools to preprocess the data and make it more manageable. We also have a team of dedicated labeling experts who are trained to work efficiently and accurately with large volumes of data.
Challenge 3: Maintaining Consistency
Consistency is key when it comes to data labeling. Inconsistent labels can lead to errors in the machine learning model, which can impact its performance. To maintain consistency, it’s important to have clear labeling guidelines and a robust quality assurance process.
At Springbord, we have a team of experts who are trained to maintain consistency in labeling. We work closely with our clients to develop clear labeling guidelines and ensure that they are followed consistently throughout the labeling process. We also have a robust quality assurance process in place to catch any inconsistencies before they impact the quality of the labeled data.
Challenge 4: Handling Complex Data
Data can come in many different forms, including text, images, and video. Each type of data presents unique challenges when it comes to labeling. For example, labeling text data requires an understanding of language and context, while labeling images and video requires an understanding of visual features and patterns.
At Springbord, we have a team of experts who are trained to handle complex data. We use a combination of automated tools and human expertise to label different types of data accurately and efficiently.
Conclusion:
Data labeling is a critical step in the machine learning process, but it’s also one of the most challenging. From ensuring accuracy and consistency to managing large volumes of data, data labeling presents several unique obstacles that can hinder the success of your machine-learning models.
At Springbord, we understand these challenges and have developed strategies to help you overcome them.
By partnering with us, you can ensure the accuracy and consistency of your labeled data and improve the performance of your machine-learning models. Contact us today to learn more about our data labeling services.