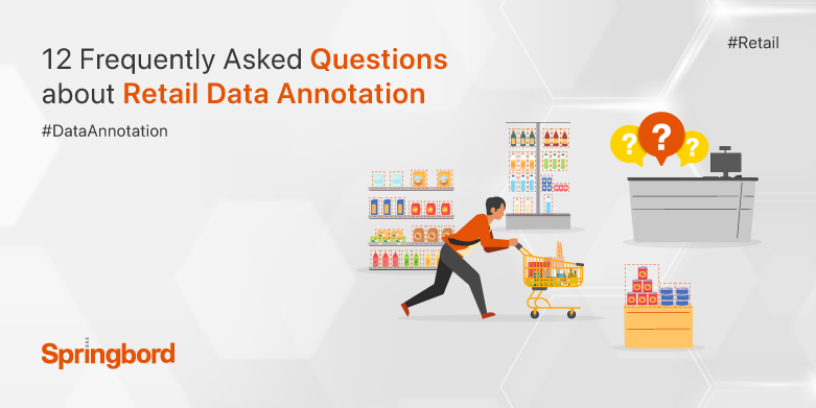
Data annotation is crucial for retail businesses, but it comes with its own set of challenges. From determining the right annotation method to managing large data sets, retailers are often faced with various obstacles. Springbord has compiled a list of the most frequently asked data annotation challenges for retail and offers expert solutions to overcome them.
As technology continues to advance and the retail industry becomes increasingly data-driven, the need for accurate and reliable data annotation is crucial. Data annotation, the process of labeling and categorizing data for machine learning and artificial intelligence, is essential for retailers to gain valuable insights and improve their operations.
However, with the vast amount of data that needs to be annotated, retailers may face several challenges when it comes to data annotation. In this blog, we will discuss some of the most frequently asked data annotation challenges for retailers and how Springbord can help.
Where do I start with my data?
One of the most common challenges when starting with data annotation for retail is knowing where to begin. One approach is to start by identifying the specific use case for the data.
For example, if the goal is to train a model for product classification, start by gathering a representative sample of product images and their associated labels. Once the data has been gathered, it can be sent for annotation.
How much data do I need, and when?
Another challenge is determining how much data is needed for annotation and when it should be done. The amount of data needed will vary depending on the specific use case, but as a general rule, the more data that is available, the better the model will perform.
Additionally, it is important to consider the timing of the annotation process, as it should be done in a way that aligns with the overall development and deployment timeline.
How do I make sure that the data I send to be annotated is a good representation of the data from production?
Ensuring that the data sent for annotation is representative of production data is crucial for the performance of the model.
One way to do this is to use a mix of different data types, such as images, text, and audio, to ensure that the model is able to handle a variety of inputs. Additionally, it is important to use data from a variety of sources, such as customer reviews and sales data, to ensure that the model is exposed to a diverse range of inputs.
How do I make sure that my data doesn’t have any bias built in?
The inherent bias in data can lead to poor model performance and unfair decisions. One way to mitigate bias is to use data from a diverse range of sources and to ensure that the data is annotated by a diverse group of annotators.
Additionally, it is important to use techniques such as data preprocessing and data augmentation to reduce bias in the data.
What parts of my training data should I have annotated first?
When deciding which parts of the training data to get annotated first, it is important to prioritize the most important and urgent use cases.
For example, if the goal is to train a model for product classification, it is likely more important to annotate images of products than images of store interiors.
Additionally, it is important to consider the availability of annotators and the overall annotation budget when making this decision.
What do I do with special cases?
Special cases can be difficult to handle during data annotation for retail. These can include items that are out of stock, or items that are not yet available for purchase. One solution is to create a separate label for these items so that they can be easily distinguished from other items.
Another solution is to create a label that indicates the item is out of stock or not yet available, and then use that label when annotating the item.
How many and what kind of labels do I need?
When it comes to data annotation for retail, the labels that are needed will vary depending on the specific data set. For example, if the data set contains clothing items, labels may include size, color, and material. However, if the data set contains food items, labels may include ingredients and nutritional information.
The quality of the labels is also important, as it can affect the accuracy of the data. To ensure high-quality labels, it may be necessary to have multiple people review the labels and make any necessary corrections.
How do I capture edge cases?
Capturing edge cases can be a significant challenge when annotating retail data. Edge cases refer to situations that are rare or unusual, but still need to be considered in order to ensure accurate and comprehensive data annotation.
For example, when annotating images of clothing, an edge case might be a product that is worn in an unexpected way, such as a scarf that is used as a headwrap. To capture these edge cases, it is important to use a variety of annotation techniques, such as manual annotation, crowd sourcing, and computer vision, to ensure that all possible scenarios are considered.
Additionally, it can be helpful to have a diverse group of annotators to ensure that different perspectives and experiences are taken into account when identifying edge cases.
How do I manage ambiguity?
Managing ambiguity is another common challenge in data annotation for retail. Ambiguity refers to situations where the meaning of data is uncertain or open to interpretation.
For example, when annotating images of products, ambiguity might arise when a product is partially obscured or when it is difficult to discern the exact product being shown.
To manage ambiguity, it is important to use clear and consistent annotation guidelines, as well as to have a thorough quality control process in place to ensure that annotations are accurate and consistent.
Additionally, it can be helpful to use machine learning and computer vision techniques to assist in the annotation process, as these technologies can help to reduce the potential for ambiguity.
How negatively impactful are errors in my data?
Errors in data annotation can have a significant impact on the accuracy and effectiveness of retail models. For example, if a product is mislabeled or an annotation is inaccurate, it can lead to incorrect predictions or recommendations.
To minimize the impact of errors, it is important to use a thorough quality control process to catch any errors before they make it into production.
Additionally, it can be helpful to use machine learning and computer vision techniques to assist in the annotation process, as these technologies can help to reduce the potential for errors.
How can I make sure my model in production is still performing as expected?
To ensure that a model in production is still performing as expected, it is important to continuously monitor and evaluate its performance.
This can be done by comparing the model’s predictions to actual data, as well as by monitoring key metrics such as accuracy and recall.
Additionally, it can be helpful to use machine learning and computer vision techniques to assist in the evaluation process, as these technologies can help to identify patterns and trends in the data that may indicate a problem with the model.
Who can I trust to annotate my training data?
Choosing a reliable and trustworthy data annotation provider is crucial for retail companies.
Springbord is a leading provider of data annotation services for retail, with a team of experienced and skilled annotators who are able to provide accurate and comprehensive data annotation.
Additionally, Springbord utilizes a variety of annotation techniques, including manual annotation, crowd sourcing, and computer vision, to ensure that all possible scenarios are considered.
Additionally, Springbord has a thorough quality control process in place to ensure that annotations are accurate and consistent, and uses machine learning and computer vision techniques to assist in the annotation process.
By choosing Springbord, retail companies can trust that their training data will be of the highest quality and that their models will perform as expected.
Conclusion:
In conclusion, data annotation for retail is a crucial process that helps businesses understand their customers and make more informed decisions.
However, there are many challenges that come with data annotation, such as handling special cases, creating high-quality labels, capturing edge cases, managing ambiguity, and minimizing the impact of errors.
Springbord, a service provider company, is here to help businesses overcome these challenges and achieve their goals with accurate and efficient data annotation.